Updated on April 8, 2024
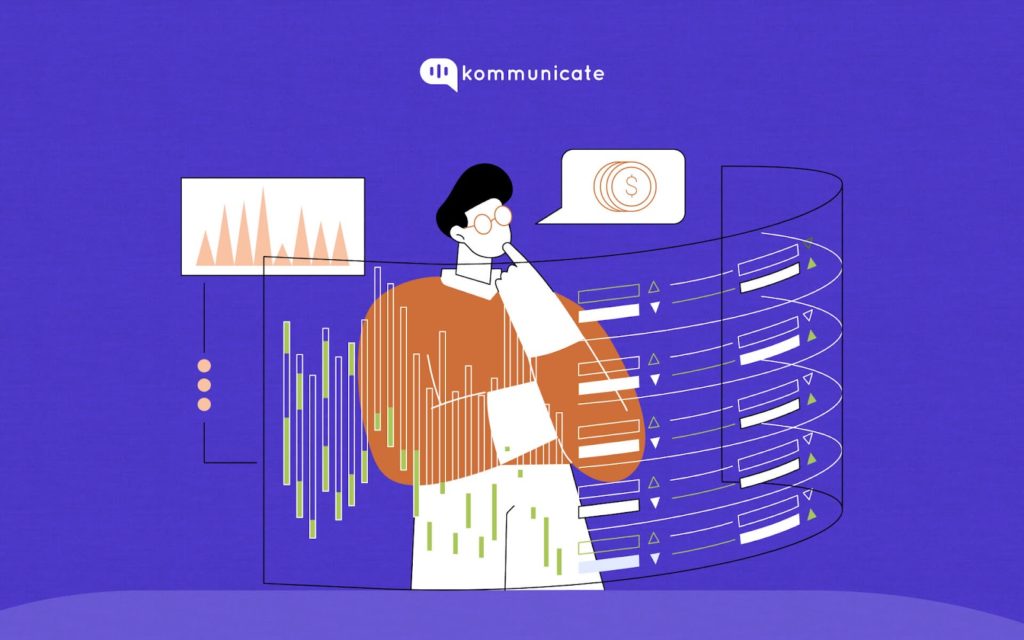
Generative Artificial Intelligence (Generative AI) can be rightly called a dynamic force that is reshaping how products are conceived, developed, and refined. For seasoned product managers steering the course of large enterprises, understanding and harnessing the power of Generative AI is no longer a luxury—it’s a strategic imperative.
What is Generative AI
At its core, Generative AI refers to systems that can create new, original content autonomously, mimicking human creativity and problem-solving. This opens a realm of possibilities, from generating realistic images to crafting human-like text, fundamentally altering the landscape of product innovation.
Key Applications and Benefits of Implementing Generative AI for Product Management
Generative AI is not merely a technological leap; it’s a paradigm shift in how we approach product development.
Undoubtedly, Generative AI is a catalyst for innovation and efficiency. Imagine a tool that not only streamlines mundane tasks but also augments the creative process, aiding in ideation and concept development.
From automating market trend analyses to generating product prototypes, Generative AI serves as a force multiplier for product managers, allowing them to focus on strategic decision-making rather than routine tasks.
This blog is not a theoretical discourse on the potential of Generative AI. Instead, it’s a pragmatic guide meticulously crafted for seasoned product managers like yourself, individuals who recognize that staying ahead means embracing innovation with a clear, actionable strategy.
Implementing generative AI is not any Plug’n’Play software that can be readily installed and used. Although it is instantly accessible, to achieve tangible results in product management, a proper implementation process is necessary.
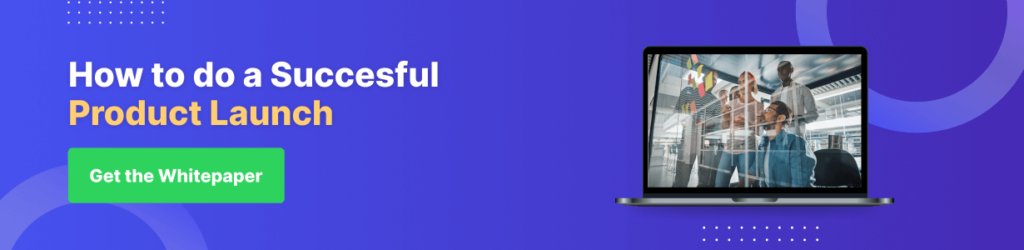
Step 1 – Assessing Organizational Readiness
The first step in generative AI implementation should be a thorough assessment of your organization’s readiness. This pivotal phase lays the foundation for successful implementation and ensures that the deployment aligns seamlessly with your business objectives and priorities.
Begin by evaluating your organization’s current AI capabilities. This includes existing AI infrastructure, data handling processes, and the proficiency of your team in working with AI technologies.
Consider the scalability and adaptability of your current AI systems, ensuring they can accommodate the integration of Generative AI without compromising existing operations.
Whether it’s enhancing product innovation, improving customer experiences, or optimizing operational efficiency, ensure that AI integration is aligned with broader business objectives. Be informed that misalignment can lead to serious inefficiencies, wasted resources, and a failure to realize the full potential of the technology.
This makes it mandatory to hold active discussions with key stakeholders to understand their expectations and identify areas within the product management lifecycle where Generative AI can deliver tangible value.
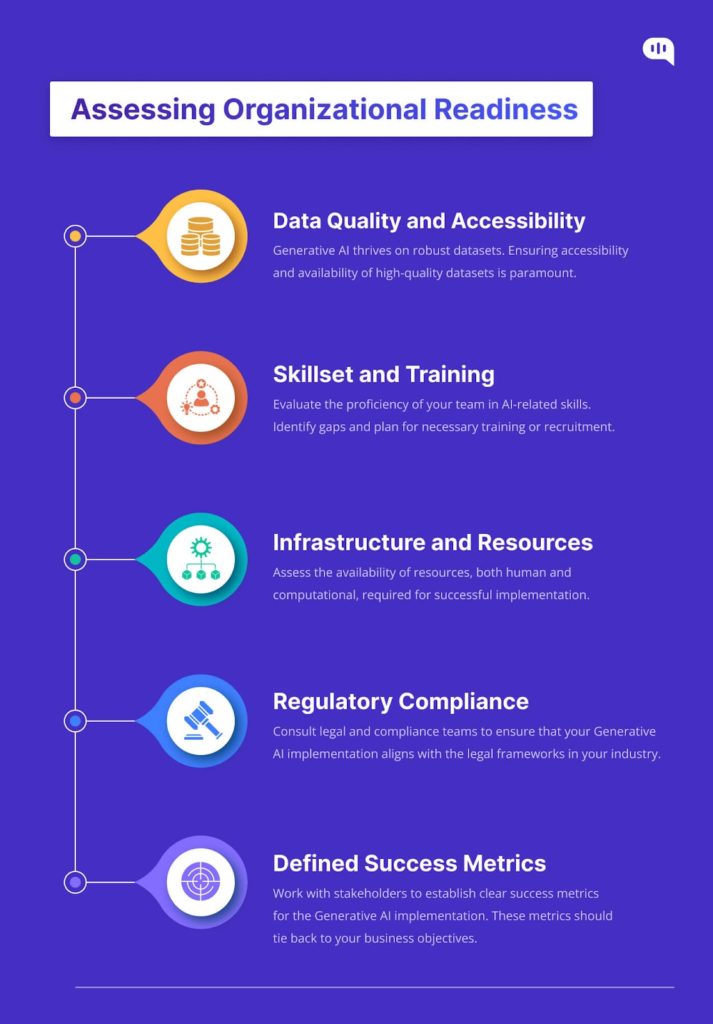
Use this checklist to check your organizational readiness for
- Data Quality and Accessibility: Generative AI thrives on robust datasets. Ensuring accessibility and availability of high-quality datasets is paramount.
- Skill set and Training: Evaluate the proficiency of your team in AI-related skills. Identify gaps and plan for necessary training or recruitment.
- Infrastructure and Resources: Assess the availability of resources, both human and computational, required for successful implementation.
- Regulatory Compliance: Consult legal and compliance teams to ensure that your Generative AI implementation aligns with the legal frameworks in your industry.
- Defined Success Metrics: Work with stakeholders to establish clear success metrics for the Generative AI implementation. These metrics should tie back to your business objectives.
By systematically evaluating these aspects, you lay the groundwork for a successful integration of Generative AI into your product management function. It also makes it easier to build a business case for the move.
Step 2 – Building the Business Case
Crafting a compelling business case for the integration of Generative AI into your product management function is a strategic endeavor that requires a clear understanding of its potential impact on your organization’s bottom line.
Top management would be curious about the efficiency gains before allocating any significant budget and resources to the cause.
This demands the product manager to painfully be specific about the pain points within the current product management processes where Generative AI can offer solutions.
Along with that provide a detailed analysis of the Return on Investment (ROI) that Generative AI is expected to deliver. This involves estimating the costs associated with implementation and contrasting them with the anticipated benefits such as time savings, cost reductions, and revenue increases.
By following these steps, you’ll be well-equipped to present a persuasive business case that not only showcases the potential of Generative AI but also instills confidence in decision-makers regarding its strategic importance for your organization’s product management function.
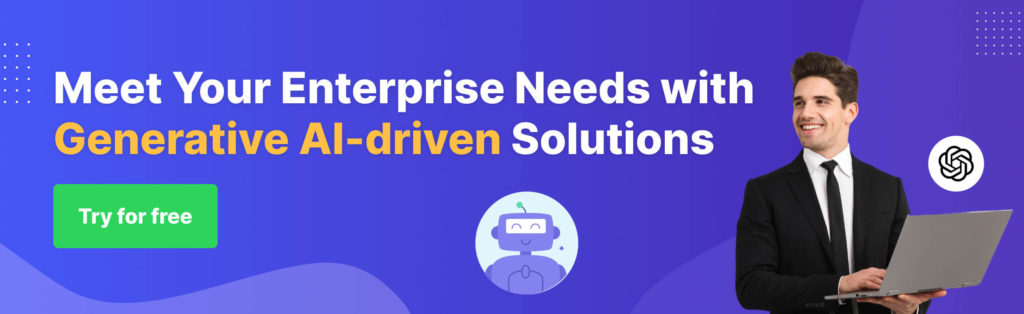
Step 3 – Assembling the Right Team
A successful implementation of Generative AI requires not only advanced technology but also a cohesive and skilled team. You will need plenty of technical talent aboard for the purpose. These include (but are not limited to):
- Data Scientists who can analyze and interpret complex data sets, develop and train models, and optimize algorithms.
- Machine Learning Engineers who can translate models into deployable solutions, work on integration with existing systems, and ensure the scalability of AI solutions.
- Domain Experts who can provide industry-specific insights to guide the customization of generative AI models for optimal results.
- Ethical AI specialists who can ensure the ethical use of AI, identify potential biases in data or models, and establish guidelines for responsible AI practices.
Be informed that Generative AI implementation is not a siloed task; it involves intertwining technical expertise with domain knowledge. Cross-functional collaboration is necessary to ensure a holistic approach to problem-solving.
This requires you, as a product manager, to break down departmental silos encouraging knowledge sharing and creativity. The diverse perspectives from different roles foster innovative solutions to challenges encountered during implementation.
By combining the strengths of individuals with varied expertise, your organization is better positioned to navigate the complexities of Generative AI and use it better for product management.
Step 4 – Data Preparation and Cleaning
The significance of clean and relevant datasets in generative AI implementation can never be overstated. It is indeed the cornerstone of the implementation process. Sourcing diverse, relevant data and employing meticulous cleaning practices are foundational steps.
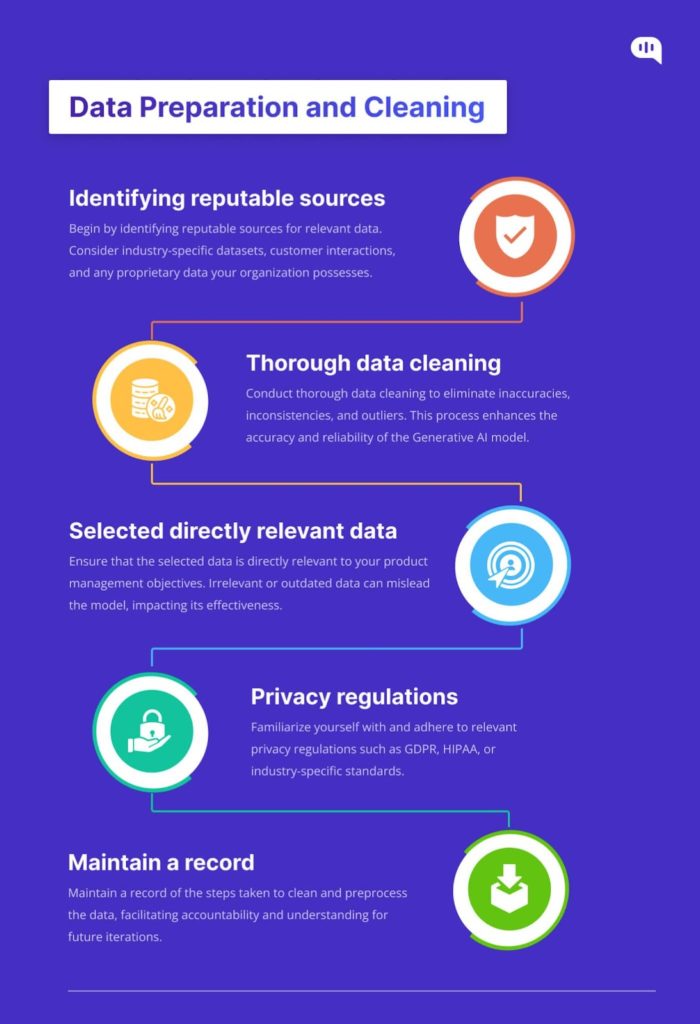
Follow these steps to ensure that data is prepared and cleaned correctly.
- Begin by identifying reputable sources for relevant data. Consider industry-specific datasets, customer interactions, and your organization’s proprietary data.
- Conduct thorough data cleaning to eliminate inaccuracies, inconsistencies, and outliers. This process enhances the accuracy and reliability of the Generative AI model.
- Ensure that the selected data is directly relevant to your product management objectives. Irrelevant or outdated data can mislead the model, impacting its effectiveness.
- Familiarize yourself with and adhere to relevant privacy regulations such as GDPR, HIPAA, or industry-specific standards.
- Maintain a record of the steps taken to clean and preprocess the data, facilitating accountability and understanding for future iterations.
Step 5 – Selecting the Right Generative Model
There are several to consider when choosing a model:
- Accuracy: Evaluate the model’s ability to produce outputs that align with the desired quality and precision for your product management goals.
- Scalability: Consider the model’s scalability, ensuring it can handle the volume and complexity of data in your product-related tasks effectively.
- Interpretability: Assess how easily the model’s outputs can be understood and interpreted, especially crucial for product managers to gain insights from the generated data.
- Training Efficiency: Examine the efficiency of the model during the training process, ensuring that it aligns with your organization’s resources and timelines for product development.
Generative Adversarial Networks (GANs) and Transformers are the two most preferred generative models in the industry today. The table below gives a comparison of how these models fare against each other based on the factors listed above.
Aspect | Generative Adversarial Networks (GANs) | Transformers |
Model | High accuracy, excels in generating realistic data. | Strong performance in capturing intricate patterns and dependencies. |
Accuracy | Challenges in scalability, especially with larger datasets. | Highly scalable, effective in handling vast datasets and complex structures. |
Scalability | Interpretability can be challenging, as GANs focus on generating data rather than explaining it. | Interpretability varies; attention mechanisms provide some interpretability, but the overall model complexity may hinder complete understanding. |
Interpretability | Training GANs can be resource-intensive and time-consuming, impacting efficiency. | Efficient training process, particularly advantageous for large-scale datasets and complex tasks. |
Step 6 – Integration with Existing Systems
Integrating Generative AI into existing product management systems presents both opportunities and challenges. A seamless integration process is essential to leverage the benefits without disrupting established workflows.
Also, it helps in:
- Minimizing downtime
- Improving user adoption
- Optimize productivity
Challenge: Legacy System Compatibility
Compatibility issues are bound to arise when integrating with legacy product management systems. Older technologies may lack the necessary interfaces for seamless integration with Generative AI.
Solution: API Standardization
Implement standardized APIs (Application Programming Interfaces) to bridge the gap between Generative AI and existing systems. This promotes interoperability and ensures a smoother integration process.
Challenge: Maintaining Data Consistency
Maintaining data consistency across integrated systems is crucial. Mismatched data formats or discrepancies can lead to errors and hinder the effectiveness of Generative AI.
Solution: Data Mapping and Transformation
Invest in tools that facilitate data mapping and transformation, ensuring data consistency between systems. This minimizes errors and maintains the integrity of information across the organization.
Challenge: Workflow Disruptions
Sudden changes in workflows can result in resistance from teams accustomed to established processes. Resistance may stem from concerns about productivity loss or uncertainty about the new technology.
Solution: Gradual Implementation
Introduce Generative AI gradually to mitigate potential disruptions. Begin with non-critical processes, allowing teams to acclimate to the technology before expanding its integration into core workflows.
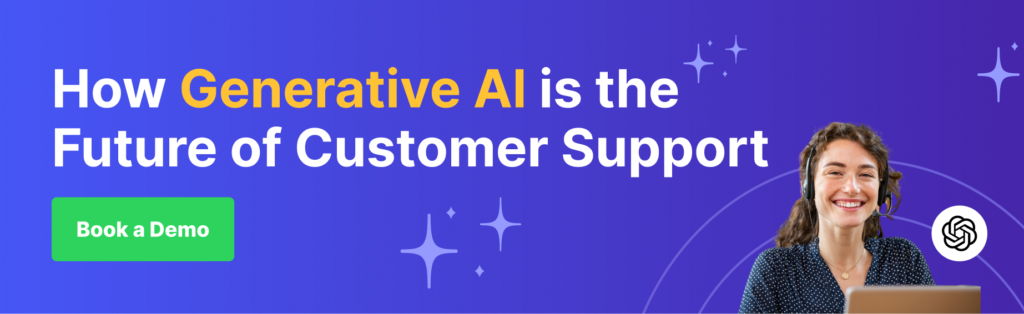
Step 7 – Testing and Quality Assurance
To ensure the reliable and ethical use of a Generative AI model, rigorous testing and quality assurance need to be used. This requires passing the model through several testing phases and strategies such as:
- Unit Testing: Used to verify the functionality of individual components within the Generative AI model.
- Integration Testing: Used to evaluate the interaction between different components and systems.
- Functional Testing: Used to validate that the Generative AI model performs as expected in real-world scenarios.
- Performance Testing: Used to measure the efficiency and responsiveness of the Generative AI model under varying workloads.
- Bias and Fairness Testing: Identify and address biases in the Generative AI model, ensuring fairness across diverse user groups.
- Ethical Testing: Assess the ethical implications of the Generative AI model, considering potential societal impacts.
In addition to software testing, it is also necessary to pass the model through Industry Standards and Guidelines on AI usage. These include:
Fairness Indicators by TensorFlow:
Leverage TensorFlow’s Fairness Indicators to quantify and visualize model performance across different subgroups, aiding in the identification of biases.
Ethics Guidelines by AI organizations:
Refer to ethics guidelines provided by organizations like the Partnership on AI (PAI) or the IEEE to ensure alignment with established ethical standards in AI development.
Algorithmic Accountability:
Adhere to algorithmic accountability frameworks, such as those proposed by the AI Now Institute, emphasizing transparency, responsibility, and user protection.
Step 8 – Monitoring and Maintenance
Implementing Generative AI is not a one-time endeavor; it requires continuous monitoring and maintenance to ensure sustained performance and adaptability to evolving requirements. Here’s why ongoing monitoring is crucial.
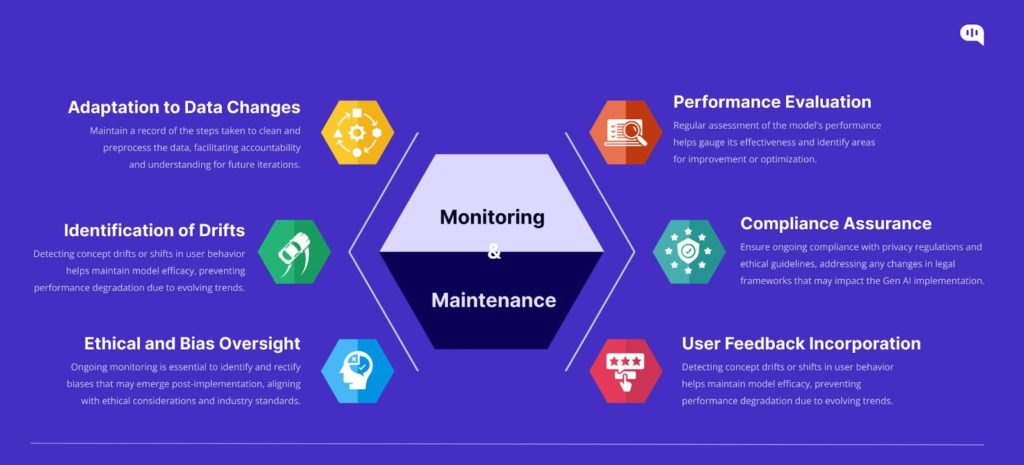
- Adaptation to Data Changes: Continuous monitoring allows the Generative AI model to adapt to changes in data distribution, ensuring relevance and accuracy over time.
- Identification of Drifts: Detecting concept drifts or shifts in user behavior helps maintain model efficacy, preventing performance degradation due to evolving trends.
- Ethical and Bias Oversight: Ongoing monitoring is essential to identify and rectify biases that may emerge post-implementation, aligning with ethical considerations and industry standards.
- Performance Evaluation: Regular assessment of the model’s performance helps gauge its effectiveness and identify areas for improvement or optimization.
- Compliance Assurance: Ensure ongoing compliance with privacy regulations and ethical guidelines, addressing any changes in legal frameworks that may impact the Generative AI implementation.
- User Feedback Incorporation: Actively incorporate user feedback into the monitoring process to address user concerns, improve user satisfaction, and enhance the user experience.
Conclusion: Transformative Potential of Generative AI in Product Development
Embracing Generative AI is not just an option—it’s a strategic imperative for staying ahead in innovation and competitiveness. As senior product managers, implementing Generative AI involves careful planning, thoughtful consideration, and continuous adaptation.
In embracing Generative AI, senior product managers like you can unlock a new era of possibilities, where data-driven creativity and strategic decision-making converge. It’s not just about adopting technology; it’s about reshaping the future of product development.
As you embark on this transformative journey, remember that the power of Generative AI lies not just in its algorithms but in its ability to amplify human ingenuity and redefine the possibilities of product management.
Manab leads the Product Marketing efforts at Kommunicate. He is intrigued by the developments in the space of AI and envisions a world where AI & human works together.