Updated on April 2, 2025
This is the era of the consumer. Everything a brand does or plans to do depends on what consumers wish to buy or see. Customization and personalized experiences are at their peak, and brands are competing with each other for consumer attention.
In addition, the existence of multiple channels has enabled countless touchpoints where users can reach and interact with. Furthermore, consumers are becoming increasingly tech-savvy, and using traditional typing methods isn’t everyone’s cup of tea either – especially accounting for Gen Z.
Considering all the variables involved in catering to a tech-savvy, contemporary consumer, AI chatbots and conversational AI solutions have become essential for businesses. Therefore, it is nearly impossible for a human to deliver the quality and level of customization expected by a consumer without leveraging NLP chatbot technology.
This is where AI steps in – in the form of conversational assistants, NLP chatbots today are bridging the gap between consumer expectation and brand communication. Through implementing machine learning and deep analytics, NLP chatbots are able to custom-tailor each conversation effortlessly and meticulously.
One of the best successful AI-powered NLP chatbot examples in use today is the one by Kommunicate.io. This advanced chatbot for customer service is special because of the conversational AI technology that induces a human warmth in every interaction with a user. Furthermore, it doesn’t require any human presence to carry a conversation successfully with a customer, making it an ideal AI chatbot for business automation.
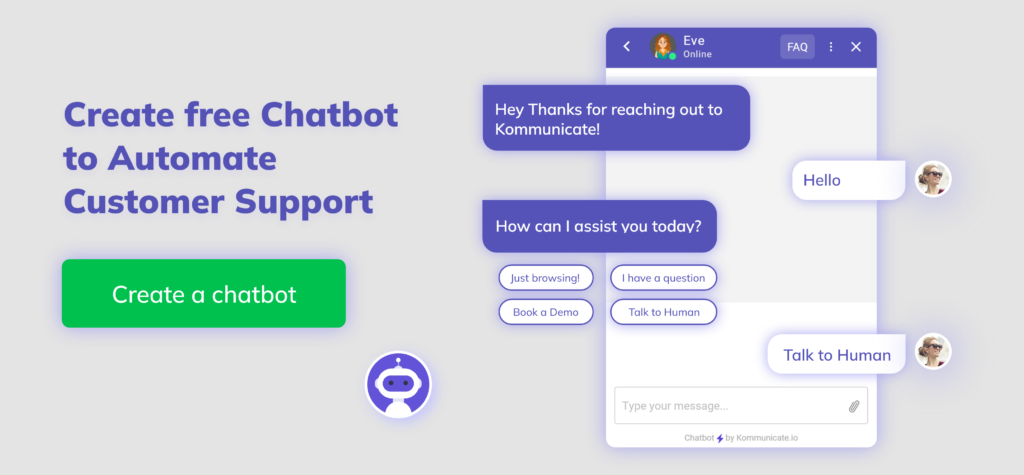
Let’s look at NLP chatbots in detail.
What is NLP Chatbot?
AI chatbots for websites are, in essence, digital conversational agents whose primary task is to interact with the consumers that reach the landing page of a business. These AI-driven chatbots help businesses enhance user engagement and provide real-time support. They are designed using artificial intelligence mediums, such as machine learning and deep learning. As they communicate with consumers, chatbots store data regarding the queries raised during the conversation. This is what helps businesses tailor a good customer experience for all their visitors.
This helped businesses free up some manpower. Today, chatbots do more than just converse with customers and provide assistance – the algorithm that goes into their programming equips them to handle more complicated tasks holistically. Now, chatbots are spearheading consumer communications across various channels, such as WhatsApp, SMS, websites, search engines, mobile applications, etc.
With the addition of more channels into the mix, the method of communication has also changed a little. Consumers today have learned to use voice search tools to complete a search task. Since the SEO that businesses base their marketing on depends on keywords, with voice-search, the keywords have also changed. Chatbots are now required to “interpret” user intention from the voice-search terms and respond accordingly with relevant answers.
A more modern take on the traditional chatbot is an AI chatbot with NLP that is equipped with programming to understand natural human speech. This is technically termed Natural Language Processing (NLP). A chatbot that can “understand” human speech and provide assistance effectively is a smart AI chatbot designed for automated customer support.
An NLP chatbot is smarter than a traditional chatbot and has the capability to “learn” from every interaction that it carries. This is made possible because of all the components that go into creating an effective NLP chatbot. Let’s take a look at these components.
Enhance customer support with AI-driven email ticketing – automate workflows and resolve queries faster with Kommunicate!
Components of NLP Chatbot
A Convincing UX
The primary purpose of an NLP chatbot is to engage with consumers. Unless the speech designed for it is convincing enough to actually retain the user in a conversation, the chatbot will have no value. Therefore, the most important component of an NLP chatbot is speech design.
This ensures that users stay tuned into the conversation, that their queries are addressed effectively by the virtual assistant, and that they move on to the next stage of the marketing funnel.
A Learning curve
Whether or not an NLP chatbot is able to process user commands depends on how well it understands what is being asked of it. Employing machine learning or the more advanced deep learning algorithms impart comprehension capabilities to the chatbot. Unless this is done right, a chatbot will be cold and ineffective at addressing customer queries.
Natural language processing
These days, consumers are more inclined towards using voice search. In fact, a report by Social Media Today states that the quantum of people using voice search to search for products is 50%. With that in mind, a good chatbot needs to have a robust NLP architecture that enables it to process user requests and answer with relevant information.
Sentiment processing
Some deep learning tools allow NLP chatbots to gauge from the users’ text or voice the mood that they are in. Not only does this help in analyzing the sensitivities of the interaction, but it also provides suitable responses to keep the situation from blowing out of proportion.
Understanding multiple languages
Needless to say, for a business with a presence in multiple countries, the services need to be just as diverse. An NLP chatbot that is capable of understanding and conversing in various languages makes for an efficient solution for customer communications. This also helps put a user in his comfort zone so that his conversation with the brand can progress without hesitation. The brand is able to collect better quality data from such a setup.
Administrative analytics
Even though NLP chatbots today have become more or less independent, a good bot needs to have a module wherein the administrator can tap into the data it collected, and make adjustments if need be. This is also helpful in terms of measuring bot performance and maintenance activities.
Robotic process automation
Smarter versions of chatbots are able to connect with older APIs in a business’s work environment and extract relevant information for its own use. They can also perform actions on the behalf of other, older systems.
Voice-enabled chatbots
These chatbots are the future. While automated responses are still being used in phone calls today, they are mostly pre-recorded human voices being played over. Chatbots of the future would be able to actually “talk” to their consumers over voice-based calls.
Let’s see how these components come together into a working chatbot.
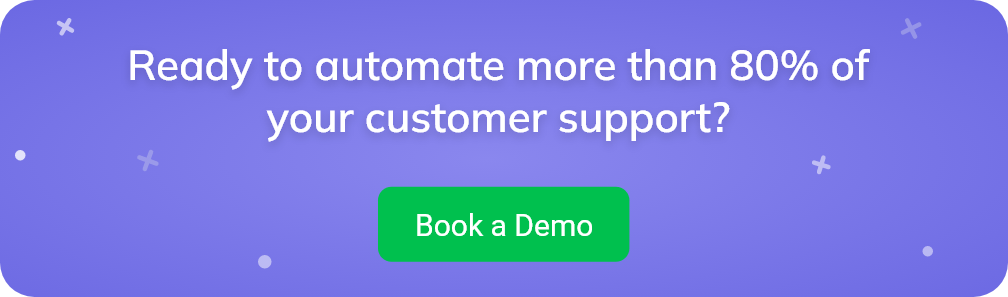
How does it actually work?
Chatbots primarily employ the concept of Natural Language Processing in two stages to get to the core of a user’s query.
When a user punches in a query for the chatbot, the algorithm kicks in to break that query down into a structured string of data that is interpretable by a computer. The process of derivation of keywords and useful data from the user’s speech input is termed Natural Language Understanding (NLU). NLU is a subset of NLP and is the first stage of the working of a chatbot.
The next step in the process consists of the chatbot differentiating between the intent of a user’s message and the subject/core/entity. In simple terms, you can think of the entity as the proper noun involved in the query, and intent as the primary requirement of the user. Therefore, a chatbot needs to solve for the intent of a query that is specified for the entity.
For example, in the user query, “When is Halloween this year?”, the intent of the user is clearly to know the date of Halloween, with Halloween being the entity that is talked about.
When a chatbot is successfully able to break down these two parts in a query, the process of answering it begins. This is where the NLP engine kicks in. NLP engines are individually programmed for each intent and entity set that a business would need their chatbot to answer.
For example, a restaurant would want its chatbot is programmed to answer for opening/closing hours, available restaurant reservations, phone numbers or extensions, etc.
Once the intent has been differentiated and interpreted, the chatbot then moves into the next stage – the decision-making engine. Based on previous conversations, this engine returns an answer to the query, which then follows the reverse process of getting converted back into user comprehensible text, and is displayed on the screens.
Why NLP chatbot?
NLP makes any chatbot better and more relevant for contemporary use, considering how other technologies are evolving and how consumers are using them to search for brands.
One of the major reasons a brand should empower their chatbots with NLP is that it enhances the consumer experience by delivering a natural speech and humanizing the interaction.
In addition, NLP for chatbots offers the following benefits:
- They are mission-critical and focused on delivering relevant information.
- It help to reduce manpower costs to the company.
- They are known to fetch higher satisfaction rates from customers.
- They are a great tool for market research and analysis in-house.
Let’s see how you can use a chatbot in your business.
How to Use Chatbots in Your Business?
Contrary to the common notion that chatbots can only use for conversations with consumers, these little smart gen AI chatbot applications actually have many other uses within an organization. Here are some of the most prominent areas of a business that chatbots can transform.
- Chatbots work phenomenally great as support in call centers. Call center requests involving straightforward tasks that don’t require critical thinking and are handled by chatbots, reducing the load on actual humans working there. An NLP chatbot can handle requests such as fetching utility bills, changing passwords, or lodging a complaint (utility services, entertainment, etc.).
- Back-end process support can easily handled by chatbots. Things such as inventory management or handling CRM come within the scope of a chatbot’s capabilities(real estate industry).
- The best of all, chatbots work as digital personal assistants that help customers speedily complete purchases (groceries, apparel apps)
How to create an NLP chatbot
Today, almost everything can be bought off the shelf. If you think that this isn’t possible for chatbots, you are wrong. Kompose offers ready code packages that you can employ to create chatbots in a simple, 1-2-3 step methodology. If you know how to use programming, you can create a chatbot from scratch. If not, you can use templates to start as a base and build from there.
🚀 Bonus: Build Your Own NLP Chatbot
Top 10 NLP chatbot platforms
Here is a comprehensive list of the top 10 NLP chatbot platforms:
Platform | Features | Price |
Lobster | Advanced conversational AI | 1500 pounds per month |
Drift | AI, revenue acceleration | Free |
Kommunicate | Custom bots, codeless bot integration | $40+p/m |
Messenger People | Engage community, manage digital assets | $25-$250+ p/m |
Agentbot | TPI, Multi-language predictive analytics | $240 |
Botscrew | Code-free development, omnichannel | $600+ |
Rapiwha | Scheduling, Instagram analytics | $0-$49+ p/m |
Botsociety | Reporting, publishing, engagement | $99-$249 p/m |
Chatfuel | NLP, designed for Facebook | $300p/m |
MobileMonkey | Lead generation, drip campaigns | $49-$149p/m |
Aivo | Scalable, real-time, text and voice | $240 p/m |
Challenges of NLP
Every innovation faces hiccups as it is developing. NLP merging with chatbots is a very lucrative and business-friendly idea, but it does carry some inherent problems that should address to perfect the technology. The first challenge that NLP faces is the problem of homonyms. Inaccuracies in the end result due to homonyms, accented speech, colloquial, vernacular, and slang terms are nearly impossible for a computer to decipher.
Additionally, while all the sentimental analytics are in place, NLP cannot deal with sarcasm, humour, or irony. Jargon also poses a big problem to NLP – seeing how people from different industries tend to use very different vocabulary.
Some of the other challenges that make NLP difficult to scale are low-resource languages and lack of research and development.
Conclusion
The day isn’t far when chatbots would completely take over the customer front for all businesses – NLP is poised to transform the customer engagement scene of the future for good. It already is, and in a seamless way too; little by little, the world is getting used to interacting with chatbots, and setting higher bars for the quality of engagement.
Artificial intelligence is all set to bring desired changes in the business-consumer relationship scene.
At Kommunicate, we are envisioning a world-beating customer support solution to empower the new era of customer support. We would love to have you on board to have a first-hand experience of Kommunicate. You can signup here and start delighting your customers right away.
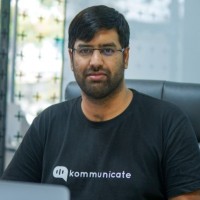
CEO & Co-Founder of Kommunicate, with 15+ years of experience in building exceptional AI and chat-based products. Believes the future is human + bot working together and complementing each other.