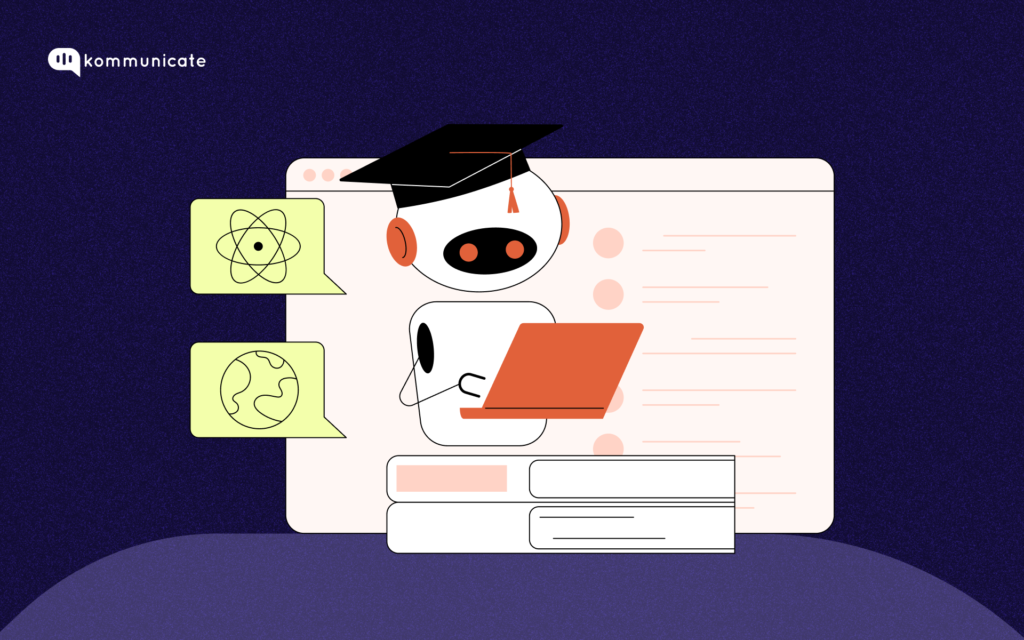
In today’s fast-paced business environment, delivering exceptional customer service is the ultimate goal for every CX leader. Customers now expect businesses to resolve queries as quickly and fluently as ChatGPT and Gemini. This blog post will explore how retrieval-augmented generation (RAG) is transforming customer service AI chatbots.
Large language models, like OpenAI’s o3, o4 mini and Anthropic’s Claude Opus 4 and Claude Sonnet 4, store vast information in their parameters (the values that define the model’s behavior). When fine-tuned (further trained) on specific tasks, these models can achieve state-of-the-art performance.
However, their ability to precisely access and manipulate their stored knowledge is still limited. So, their performance falls behind specialized models designed specifically for customer service tasks that require a lot of customized knowledge.
It’s hard to trace pre-trained models’ knowledge sources, and updating them with new information remains unsolved.
This is where Retrieval-augmented generation (RAG) comes in, making AI chatbots for customer service smarter and more accurate.
Before we start, here are some related blogs we think you might be interested in:
- Unleashing The Power Of Gen AI For Your Excel Data
- 12 Customer Service Challenges You Need To Watch Out For in 2024
- How AI Is Reshaping The Help Center In 2024
What is Retrieval-Augmented Generation (RAG)?
Retrieval-augmented generation (RAG) improves a language model’s responses by checking an external knowledge base for accurate information before generating an answer.
Retrieval-based models excel at finding relevant information from large databases or knowledge bases, while generation-based models specialize in generating natural language responses based on the input.
Traditional retrieval-based models rely solely on finding and returning pre-existing information, limiting their ability to provide tailored responses or handle complex queries.
On the other hand, generation-based models can generate human-like responses but may lack factual accuracy or coherence, especially for specialized domains.
RAG combines the strengths of both approaches, leveraging the retrieval model to gather relevant information from a knowledge base and feeding it to the generation model to produce a coherent and contextually appropriate response.
How RAG Works in Customer Service Chatbots
The RAG process in customer service chatbots involves the following steps:
- Query Understanding: The customer’s query is analyzed and processed to extract key information and intent.
- Information Retrieval: The retrieval model searches the knowledge base for relevant passages or documents related to the query.
- Context Generation: The retrieved information is combined with the original query to provide the generation model with the necessary context.
- Response Generation: The generation model uses context and language understanding capabilities to generate a natural response tailored to the customer’s query.
- Response Refinement: The generated response may undergo additional processing or filtering to ensure relevance, coherence, and adherence to customer service guidelines.
Here’s an example of RAG in action:
Customer Query: “I received a damaged product, and I’m not sure how to return it. What’s the process for returns and exchanges?”
RAG Workflow:
- The system processes the query to identify the intent: “product return/exchange process.”
- The retrieval model searches the knowledge base and finds relevant information on the company’s return and exchange policies.
- The context (query + retrieved information) is provided to the generation model.
- The generation model produces a response: “Thank you for reaching out. If you’ve received a damaged product, you can initiate a return or exchange by following these steps: [detailed instructions]. Please let me know if you have any further questions or need assistance with the process.”
- The AI reviews the response for clarity and accuracy before sending it to the customer.
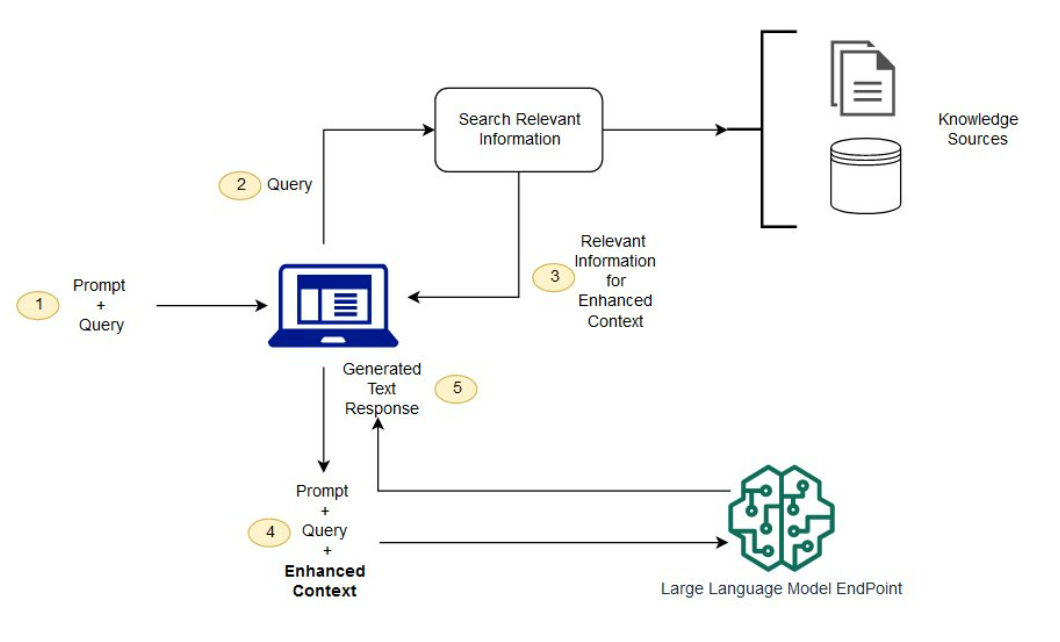
Benefits of RAG for Customer Service Chatbots
Implementing RAG in customer service chatbots offers numerous benefits, including:
- Improved Accuracy and Relevance: By leveraging both retrieval and generation capabilities, RAG chatbots can provide more accurate and relevant responses, reducing frustration and increasing customer satisfaction.
- Enhanced Ability to Handle Diverse and Complex Queries: RAG chatbots can understand and respond to a wide range of queries, from simple requests to complex, multi-faceted questions, thanks to their ability to retrieve and synthesize information from various sources.
- Better Customer Engagement: With their natural language generation capabilities, RAG chatbots can engage customers in a more human-like manner, fostering better relationships and increasing brand loyalty.
- Scalability and Efficiency: RAG chatbots can handle high volumes of customer queries simultaneously, reducing wait times and improving overall efficiency.
Success Story: ETEAM a software development company built their own LLM powered AI chatbot with RAG. They integrated the AI chatbot with Slack for employees to ask about company values, time-off, and HR queries.
Implementing RAG in Your Customer Service Strategy
To integrate RAG technology into your customer service strategy, follow these steps:
- Evaluate Your Existing Knowledge Base: Ensure your knowledge base is comprehensive, up-to-date, and well-structured to enable efficient retrieval.
- Select the Right RAG Architecture: Choose an RAG architecture that aligns with your business requirements, considering factors like domain complexity, data privacy, and scalability needs.
- Train and Fine-Tune the Models: Train the retrieval and generation models on your domain-specific data and fine-tune them to improve performance.
- Integrate with Your Customer Service Platform: Incorporate the RAG chatbot into your existing customer service infrastructure, such as live chat, email, or social media channels.
- Monitor and Continuously Improve: Continuously monitor the chatbot’s performance with LLM observability, gather feedback, and make necessary adjustments to improve accuracy and relevance.
Potential challenges in implementing RAG include data quality issues, model biases, and ensuring privacy and security. However, following best practices and working with experienced AI experts can help mitigate these challenges.
Future of RAG in AI Chatbots For Customer Service
The future of RAG in AI chatbots looks promising as the technology continues to evolve and mature. Emerging trends and advancements include:
- Multi-Modal RAG: Incorporating multiple modalities, such as images, videos, and audio, to enhance the chatbot’s understanding and response capabilities.
- Continuous Learning and Adaptation: RAG chatbots that can learn and adapt in real-time, improving their knowledge and performance based on customer interactions and feedback.
- Personalization and Context Awareness: Chatbots that can understand and adapt to individual customer preferences, histories, and contexts for more personalized and relevant responses.
Over the next five years, we can expect to see RAG technology become more widely adopted in AI customer service, with chatbots becoming increasingly intelligent, natural, and capable of handling complex queries with ease.
Automate customer queries, streamline support workflows, and boost efficiency with AI-driven email ticketing from Kommunicate!
Conclusion
Retrieval-Augmented Generation (RAG) is a revolutionary approach that combines the strengths of retrieval-based and generation-based models to create more accurate, relevant, and engaging AI chatbots for customer service. By leveraging both retrieval and generation capabilities, RAG chatbots for customer service can understand and respond to diverse queries, improve customer satisfaction, and enhance overall efficiency.
As businesses strive to provide exceptional customer experiences, adopting RAG technology in their customer service strategies can offer a significant competitive advantage. Don’t hesitate to contact us for a demo or consultation on how RAG can transform your customer service operations.
FAQs
RAG chatbots can benefit a wide range of industries, including retail, finance, healthcare, and technology, where customers have diverse and complex queries. Industries with extensive knowledge bases and a need for accurate and personalized responses can greatly benefit from RAG technology.
RAG architectures can be designed to ensure data privacy and security by implementing strict access controls, encryption, and anonymization techniques. Additionally, the retrieval model can be trained on a curated knowledge base, minimizing the risk of exposing sensitive information.
The return on investment (ROI) of implementing RAG in customer service can be substantial. By improving customer satisfaction, reducing response times, and increasing operational efficiency, businesses can expect to see cost savings, increased customer retention, and revenue growth. However, the exact ROI will depend on factors such as the scale of implementation, industry, and existing customer service infrastructure.
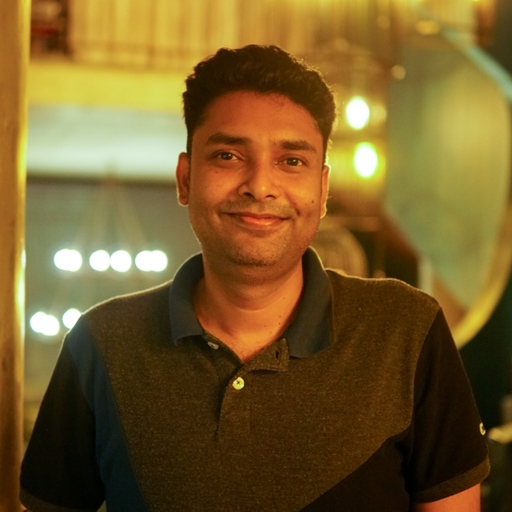
As a seasoned technologist, Adarsh brings over 14+ years of experience in software development, artificial intelligence, and machine learning to his role. His expertise in building scalable and robust tech solutions has been instrumental in the company’s growth and success.