Updated on April 4, 2025
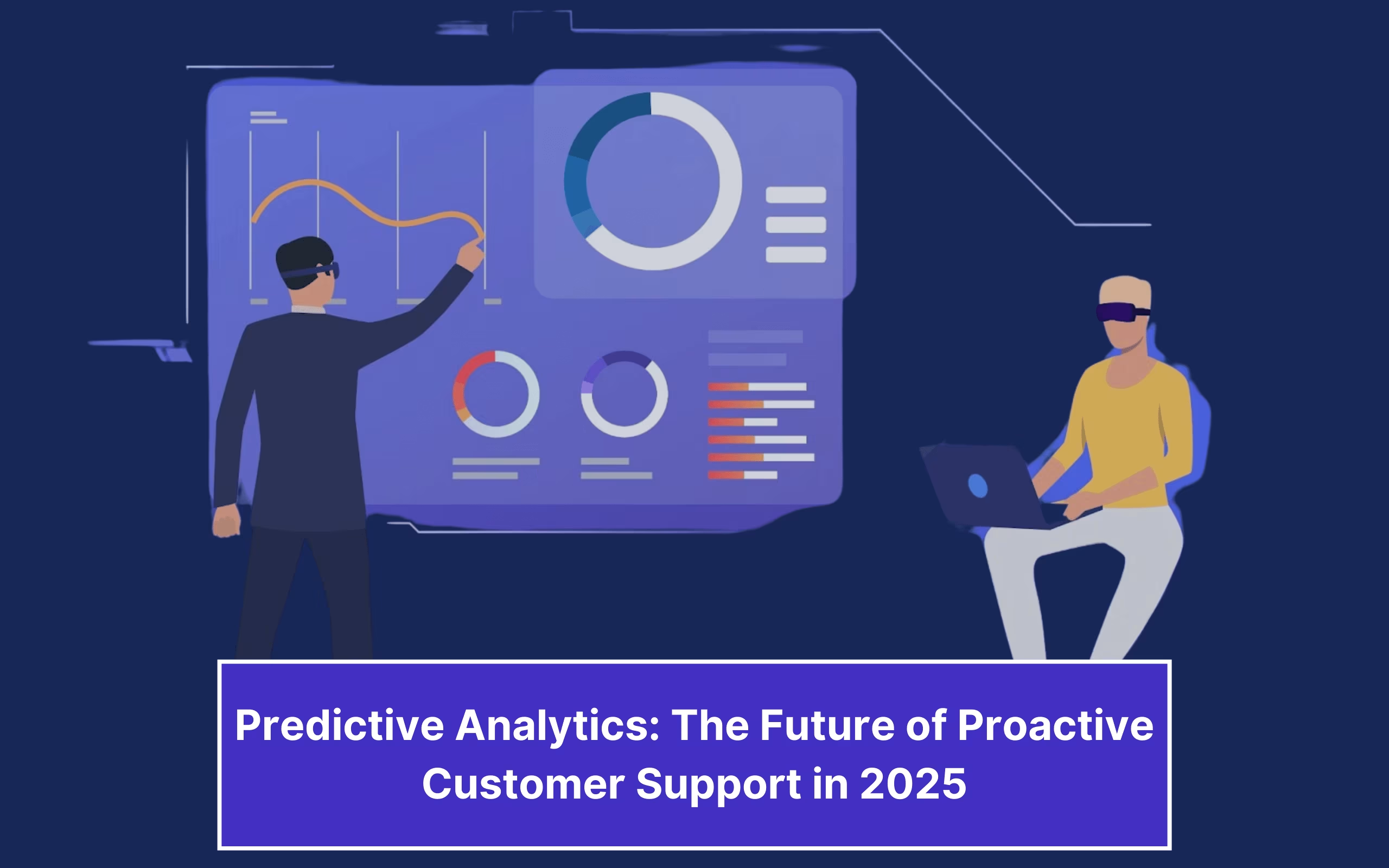
You’ve been there, waiting for a support response, repeating your issue, hoping someone figures it out soon. Traditionally, businesses relied on reactive customer service, which is waiting for customers to report issues before acting.
In 2025, the paradigm has shifted. Proactive customer support is becoming the new standard. Powered by technology, especially predictive analytics, companies can now identify potential issues before they escalate. The outcome? Fewer complaints, quicker resolutions, and a deeper understanding of customer intent.
According to a report by McKinsey, businesses implementing predictive customer support have seen operational efficiency of up to 20-30% and a 10-15% boost in customer satisfaction scores.
What is Predictive Analytics in Customer Support?
Let’s start with the basics. Most of us are used to reactive customer service—something breaks, you reach out, and only then does support step in. It works, but it’s slow.
Now, imagine the opposite. Imagine your support team reaching out before you even realize something’s off.
That’s the idea behind proactive customer service. And predictive analytics is what makes it possible.
By analyzing both historical and real-time data, predictive analytics helps businesses anticipate what customers might need next.
Here’s how proactive and reactive services stack up:
Reactive Customer Service | Proactive Customer Service |
Problems are fixed only after they’re reported. | Problems are spotted and handled before they surface. |
The customer makes the first move. | The company reaches out first. |
Takes up the customer’s time and patience. | Saves time and builds trust. |
Often relies on email or phone support. | Uses live chat, AI tools, and in-app nudges. |
Most companies still default to the reactive model. It’s simpler. But if you aim for a standout customer experience, proactive is the way forward. It takes more planning, data, and smarter tools—but the payoff is huge.
That’s where predictive analytics comes in. It turns support teams from problem-solvers into problem-preventers. From a cost center into a function that adds real value.
What Makes It Work?
A few key technologies work together behind the scenes:
- Artificial Intelligence (AI): Handles the decision-making logic and keeps the system sharp.
- Machine Learning (ML): Learns from new data to make better predictions over time.
- Big Data: Pulls in information from different sources—support tickets, user behavior, and app usage.
- Natural Language Processing (NLP): Understands tone, intent, and emotion from text-based conversations.
- Real-Time Analytics: Responds instantly, so support isn’t a step behind.
The Role of Predictive Analytics in Anticipating and Preventing Customer Issues
Customers aren’t all that different. At the heart of it, people want attention, care, and connection. Predictive analytics taps into that instinct— helping businesses anticipate needs, not just respond to complaints.
It’s not just about solving problems anymore. It’s about creating moments customers remember—because the brand showed up when it mattered.
As McKinsey puts it:
“Today’s consumers do not buy just products or services – more and more, their purchase decisions revolve around buying into an idea and an experience.”
And here’s the best part—customers don’t just remember good service. They talk about it. They trust it. They come back for it.
Why Predictive Analytics is Crucial for Customer Support
Customer expectations are rising. They want quick help, thoughtful support, and most importantly, to feel understood. Predictive analytics allows businesses to deliver just that.
Let’s explore how it creates long-term value:
1. Increases Customer Retention
According to research, 87% of customers want companies to contact them proactively. When brands take the first step, it shows care, attention, and respect for the customer’s time. Even better, 73% of customers who were contacted proactively felt a positive shift in how they viewed the brand. Eventually, this improves the retention rate.
2. Helps Acquire New Customers Through Word of Mouth
A satisfied customer will share their experience with at least three others, which can lead to new business. According to reports, word of mouth drives $6 trillion in annual consumer spending, accounting for 13% of all consumer sales.
3. Reduce Unnecessary Calls and Save Everyone Time
Support teams often deal with avoidable issues. According to a study by Sabio and the Customer Contact Association, 25% to 40% of all calls to UK contact centers are avoidable.
Most of these stem from unclear instructions, missing FAQs, or hard-to-find links. Predictive analytics helps spot these common blockers and offers support before they become tickets—saving customers and agents valuable time.
4. Protects and Enhances Brand Image
Customers talk about their experiences—especially online. If their issue goes unresolved or support feels absent, it can hurt your brand’s reputation. A known example is Dell Hell, where a customer’s blog about poor service gained traction and caused major damage to the company’s image.
5. Identifies and Fixes Pain Points Early
Many customers turn to the phone simply because they can’t find what they need online. A study by the Corporate Executive Board found that 57% of inbound calls could be avoided if customers could locate answers on the company’s website.
6. Saves Valuable Customer Time
Customers don’t want to search endlessly for answers. If your system can spot what they might struggle with and offer help instantly—whether it’s a chatbot, guide, or FAQ—it saves them time.
Predictive analytics provides support by analyzing behavior and triggering help when needed. This frees the customer to focus on exploring your product or deciding rather than troubleshooting.
7. Reduces Pressure on Support Agents
When support is only reactive, your team is constantly putting out fires. That leads to burnout, longer wait times, and dropped service quality.
Predictive analytics reduces this pressure. Solving common problems in advance means fewer tickets to get to your agents. This gives them more time to focus on high-impact queries and offer better human support.
8. Creates a Smarter, Self-Improving Support System
Predictive systems keep learning. Every interaction adds new data, helping the system improve at spotting trends, offering suggestions, and improving speed and accuracy.
Over time, your support becomes faster, more personalized, and less dependent on manual input. That means fewer repetitive tasks, quicker resolutions, and more consistent channel experiences.
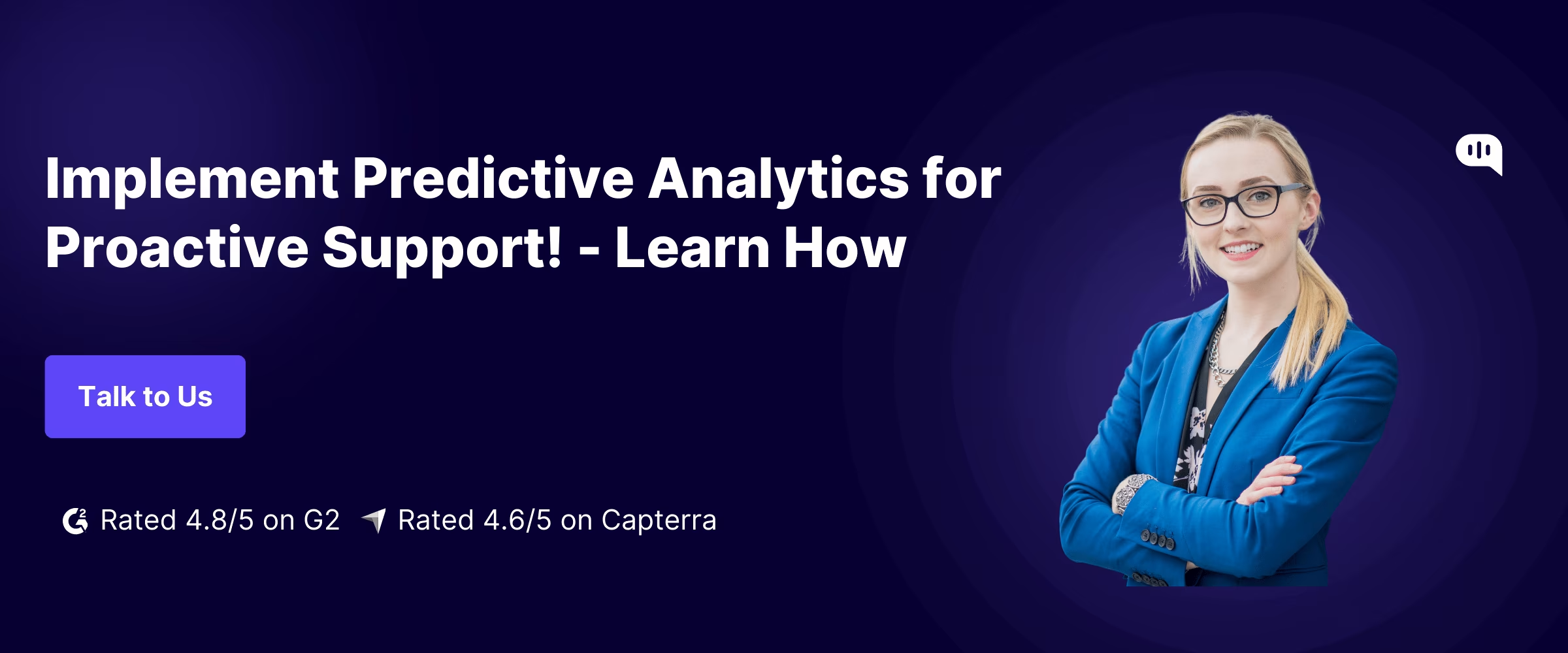
How Predictive Analytics Works in Customer Support
Predictive analytics might sound technical, but at its core, it’s about listening better, responding faster, and preventing issues before they grow.
It Starts with the Right Data
Companies first need the right kind of information to understand what customers need—or what they might struggle with. This data comes from various everyday interactions:
1. Customer Interactions
Every chat message, email, or phone call holds valuable clues. Support platforms collect this data to identify patterns—like repeated complaints, common product questions, or topics that confuse users.
Over time, these patterns help systems recognize early signs of trouble, such as a sudden spike in queries about a specific feature or service.
2. Feedback, Surveys, and Social Media Sentiment
Surveys and feedback forms provide direct insights into how customers feel. However, not all feedback is formal—people often share their experiences on social media or review platforms.
Sentiment analysis tools scan these sources for emotional cues. Even if a customer hasn’t raised a complaint directly, their tone online—words like “disappointed,” “slow,” or “frustrated”—can indicate a need for attention.
3. Transaction History and Behavioral Analytics
Your customer’s actions can say a lot—without them ever saying a word. Predictive systems can spot both interest and hesitation by looking at purchase history, time spent on different features, or abandoned checkouts.
For example, if someone keeps exploring an upgrade page but doesn’t purchase, the system might prompt a tailored offer or connect them with support for guidance.
Then Comes the Prediction Layer
Once the data is collected, predictive models go to work. They use past trends and current behavior to guess what will happen next—and help teams prepare in advance.
4. Pattern Recognition from Historical Data
Machine learning algorithms scan past support data to identify what usually happens after specific events. For instance, if login errors spike after a new update, the system learns to watch for that and raise a flag the next time.
5. AI-Driven Forecasts
These systems don’t just spot what’s wrong—they predict what might go wrong. For example, they can estimate higher ticket volumes during festive sales, or identify customer segments more at risk of cancelling their subscription.
6. Sentiment Analysis Using NLP
Natural Language Processing (NLP) tools read between the lines. They scan customer language in chats, emails, and reviews to understand tone and emotion—not just words.
Real-World Applications & Case Studies
Leading brands use predictive analytics and proactive customer service to enhance customer experiences daily.
Let’s take a closer look at some real-world examples:
1. Amazon
Amazon uses predictive analytics to stay ahead of potential shipping issues. The system can spot expected delays in advance by analyzing warehouse congestion, weather forecasts, and delivery patterns. Instead of waiting for complaints, Amazon notifies customers proactively—sometimes even offering expedited shipping or credits.
2. Verizon
Verizon analyzes live network traffic and hardware data in the telecom space to detect early signs of service disruptions. Their system often spots outages before customers do—and sometimes fixes them before a complaint is made.
3. Netflix
Netflix uses behavioral analytics to predict what content users will enjoy. Their recommendation engine isn’t just a nice feature—it keeps users engaged and improves retention. But they go even further: if buffering issues occur, Netflix automatically adjusts video quality or suggests offline viewing, all without the user needing to ask.
4. Banking
Banks rely heavily on AI to spot unusual account activity. Predictive systems trigger instant alerts if a transaction looks suspicious—even before the customer notices. These real-time fraud prevention measures reduce user stress and reduce lengthy claim processes.
5. Slack
Slack uses its intelligent assistant, Slackbot, to onboard new users personally and engagingly. When someone signs up, Slackbot initiates a friendly conversation, offers guidance, and connects users with others with similar interests or use cases.
6. Apple
Apple is known for its sleek products and thoughtful customer engagement. A great example is their “Shot on iPhone” campaign. Instead of waiting for feedback, Apple invited users to share their creativity by capturing moments with their iPhones. Selected entries were then featured on Apple’s social media platforms.
Implementing Predictive Analytics in Customer Support
Thinking about adding predictive analytics to your support strategy? Great choice.
Here’s a step-by-step breakdown to help you get started:
1. Start with Clean and Consistent Data
Your system is only as good as the data it runs on. That means ensuring your data is accurate, updated, and securely stored. Poor data leads to poor predictions, so invest time cleaning and structuring it well.
Following data privacy laws like GDPR, CCPA, or India’s DPDP Act is also important. Having strong data governance from the start helps you scale without compliance headaches.
2. Integrate the Right Tools Across Systems
Choose AI-powered platforms like Salesforce Einstein, Zendesk AI, or Freshdesk, and ensure they integrate seamlessly with your CRM, product databases, website analytics, and support ticketing tools.
3. Build a Cross-Functional Team
Predictive analytics needs different skill sets working together. Data scientists build the models, IT maintains the infrastructure, and support leads translate outputs into actions that improve the customer experience.
4. Use Customer Feedback to Fine-Tune the System
Regular feedback helps shape smarter predictions. Use surveys, feedback forms, live chat ratings, or support call notes to gather insights. If a customer points out a recurring issue, fix it at the source and train your model with that data.
5. Create Smart Self-Service Options
Predictive analytics helps identify where customers commonly get stuck. Use that insight to create helpful resources and place them in visible spots like your chat widget, help center, or dashboard.
6. Enable Real-Time Support Triggers
Monitor how users behave on your website or app. If someone lingers on a page too long, revisits a pricing section multiple times, or abandons a checkout process, set up live chat triggers to offer help at the right time.
Use tools like Google Analytics or Hotjar to analyze user behavior and create well-timed, relevant messages.
7. Listen to What Customers Are Saying Online
Customers often talk about your brand on social media—even when they don’t tag you. Use sentiment analysis tools to monitor public feedback and respond quickly when something goes wrong.
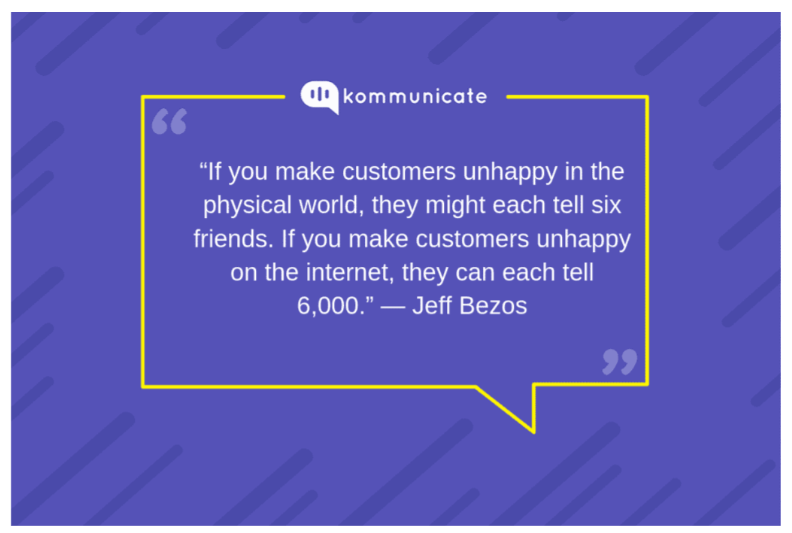
8. Add a “Recommended For You” Experience
Using customer behavior, predictive systems can suggest the next step—whether that’s a product, feature, or support article. This saves customers time and keeps them engaged without forcing them to search independently.
9. Launch an Email Newsletter That Adds Value
Newsletters are a simple way to keep customers in the loop. Use predictive insights to personalize content—like tips related to their plan, tutorials for features they use, or new product updates that match their interests.
10. Keep the System Evolving
Predictive models aren’t a one-time setup. Customer behavior changes, and so should your models. Regularly review model performance, update data sets, and audit outputs for accuracy.
Challenges & Considerations
While predictive analytics offers great potential, it’s not without its challenges. Businesses must carefully navigate a few important areas to make it work at scale and with integrity.
Data Privacy and Compliance
Predictive systems rely heavily on collecting and analyzing customer data. But more data means greater responsibility. Businesses must follow data protection laws like GDPR (Europe), CCPA (California), and DPDP (India), which set clear rules on how personal data should be handled.
To stay compliant, companies need to:
- Get clear customer consent before collecting data.
- Use only the data that’s truly necessary (data minimization).
- Anonymize or encrypt sensitive information wherever possible.
Customer Trust and Transparency
There’s a thin line between helpful and intrusive. If a brand personalizes too much or uses data without a clear explanation, it can make customers uncomfortable.
The solution is transparency. Businesses should explain what data is used, why it’s being used, and how it benefits the customer. Giving users control—such as opting out of certain tracking—goes a long way in building long-term trust.
Scalability and Integration
What works well for one team might not scale across regions, teams, or product lines. Predictive systems need to grow with the business. That means handling more users, data, and touchpoints without breaking.
One major challenge? Legacy systems. Older tools and disconnected platforms can make integration slow and complex.
To manage this, companies should:
- Use modular solutions that plug into existing systems.
- Choose platforms that support open APIs.
- Plan for scalable architecture from the start.
What’s Next: Future Trends in Predictive Customer Support
As predictive technology evolves, we’ll see more advanced, seamless, and customer-friendly support experiences. Here’s what’s around the corner:
Smarter, AI-Driven Self-Service
Chatbots are getting smarter. In 2025, many will be equipped with predictive capabilities to anticipate what customers need before they ask. These bots will understand context, handle multi-step conversations, and offer solutions based on real-time insights.
True Omnichannel Support
Customers don’t stick to one channel. They move between chat, email, calls, and social media. Future predictive systems will connect these interactions, creating a unified view of the customer.
Hyper-Personalization in Real Time
The next level of personalization isn’t just about using someone’s name. It’s about adapting tone, content, and recommendations based on live behavior, preferences, or mood.
Imagine a support tool that knows when a user is frustrated and switches to a gentler tone or offers a shortcut based on their past activity. This kind of real-time personalization builds stronger connections and encourages loyalty.
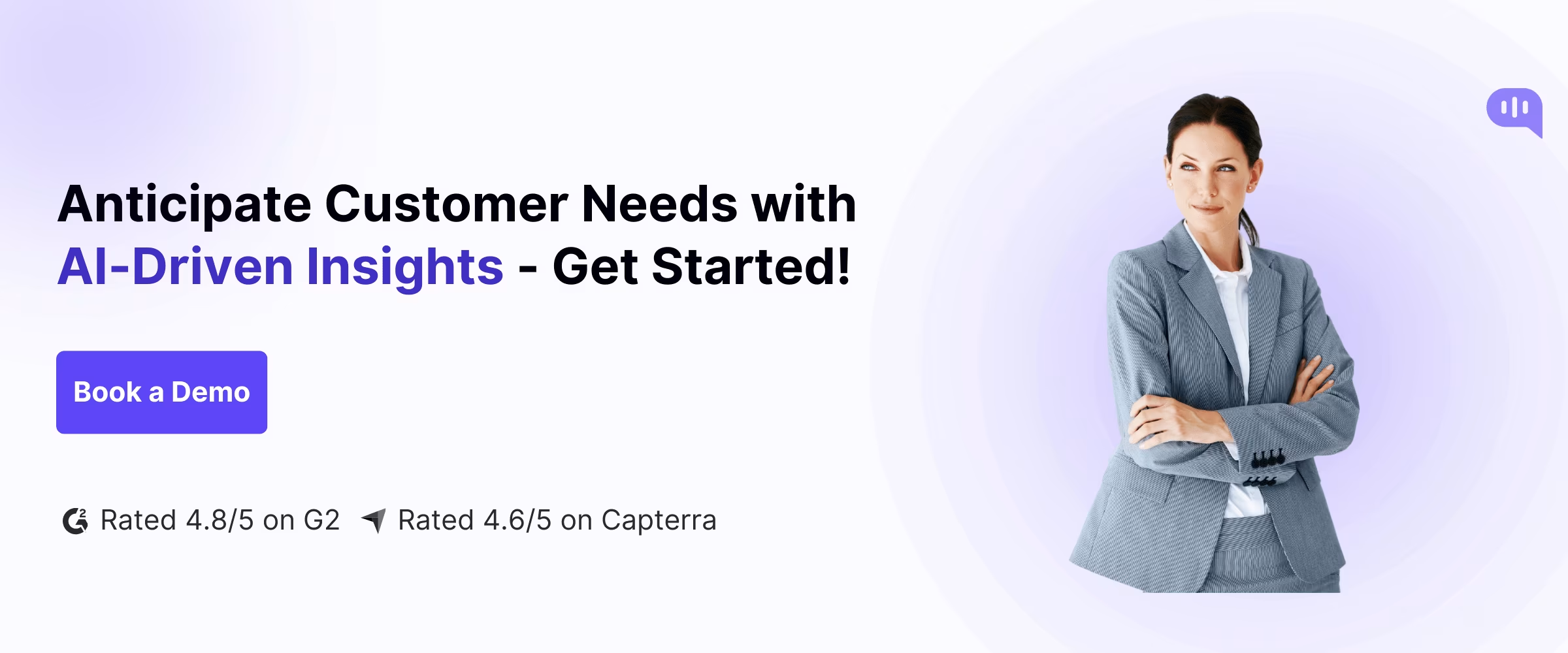
Responsible AI and Governance
As AI becomes more central to decision-making, businesses must implement safeguards. Expect more companies to adopt AI governance frameworks—with clear rules around fairness, transparency, and bias detection.
Empowered Support Agents
AI won’t replace your support team but will make their work easier. Tools that auto-summarise tickets, tag conversations, and suggest replies will reduce manual work and free up agents to focus on what matters: empathy, problem-solving, and personal attention.
Parting Thoughts
Predictive analytics is transforming how companies approach customer support in 2025. The shift from reactive responses to proactive engagement means businesses are no longer just solving problems. With the support of technologies like AI, machine learning, big data, and real-time analytics, brands across industries—from e-commerce to banking—are seeing measurable results. These include higher satisfaction scores, lower support costs, and improved operational efficiency.
But like any major change, implementation comes with its challenges. Data quality, ethical AI usage, and scalable integration are as important as the technology.
Kommunicate is a leading example with a monthly newsletter to its users. The newsletter covers new features, chatbot integration tutorials, and industry trends. This helps customers stay informed, continue learning, and experience proactive service throughout their journey.
As the Head of Growth, Marketing & Sales, Yogesh is a dynamic and results-driven leader with over 10+ years of experience in strategic marketing, sales, and business development.