Updated on April 11, 2025
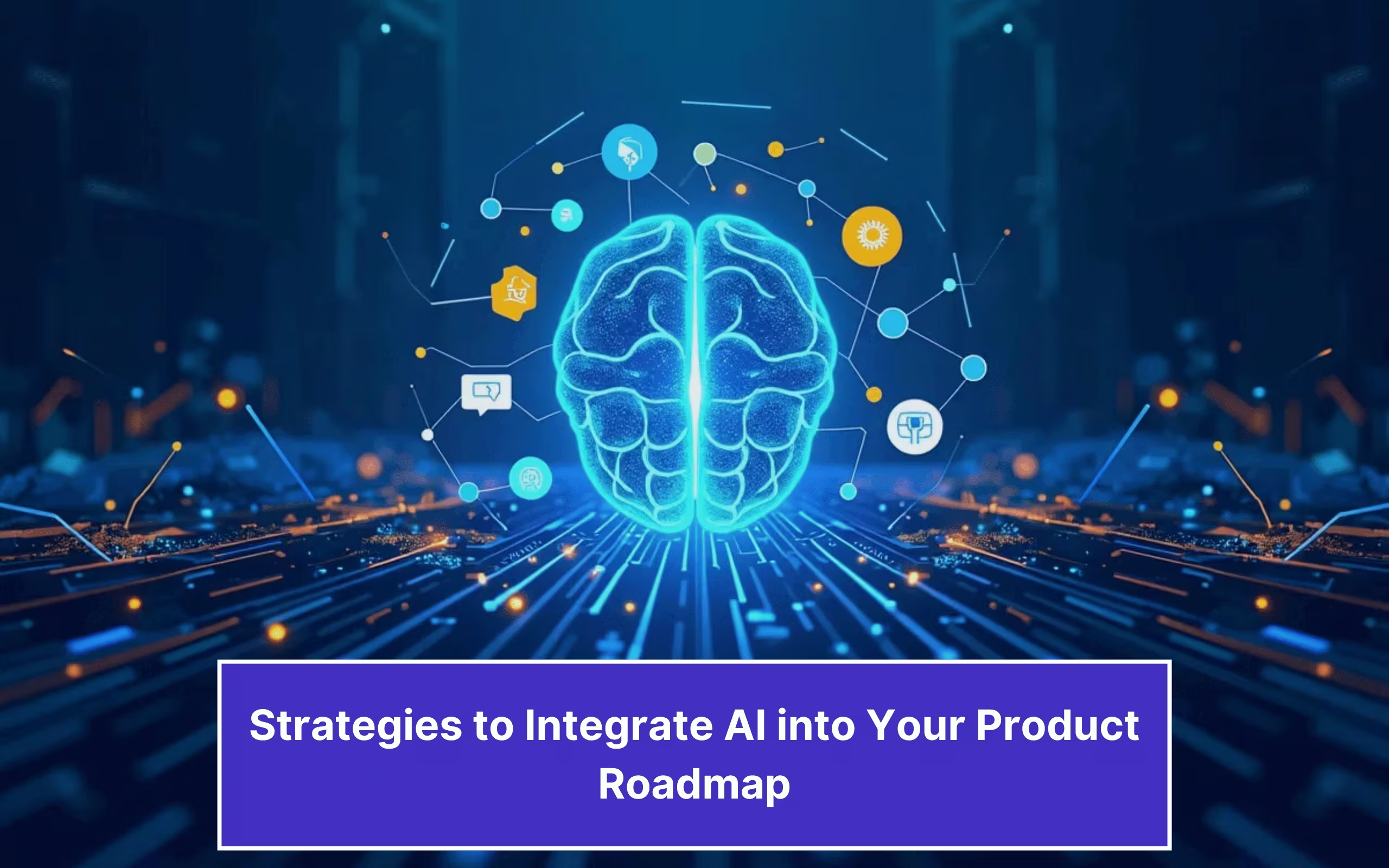
Advanced intelligence technology rapidly modifies product management solutions. The latest McKinsey AI survey reveals that 65% of organizations use generative AI in their operations. This results in business organizations boosting operational efficiency and creating personalized customer interactions.
Businesses must approach AI integration through specific methods which vary from one case to another. This article will address the key challenges and solutions for integrating AI product management. It will cover:
- Understanding AI Product Management
- Key AI Technologies Driving Innovation
- How AI Enhances Customer Experience
- 10 Strategies for Successfully Integrating AI
- Challenges and Risks in AI Product Management
By the end, you’ll have a clear framework for adopting AI in your product roadmap.
Understanding AI Product Management
AI product management functions beyond simple artificial intelligence integration in products. It represents a fundamental business change that enhances decision systems while optimizing operational processes. Large enterprises harness generative AI to automate workflows, generate data-driven insights, and deliver adaptive solutions that evolve with user needs. The path to success requires business entities to understand leading artificial intelligence technologies for innovation and their influence on customer experience.
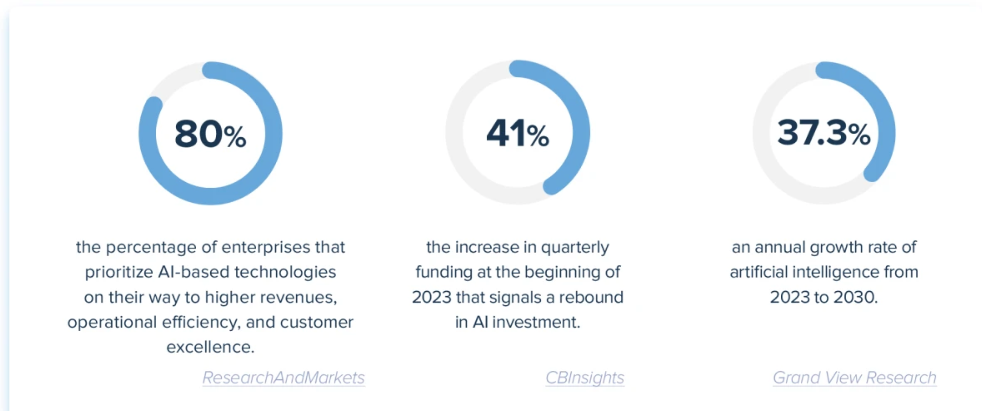
Key AI Technologies Driving Product Innovation
Implementing AI product management depends on fundamental technology elements that drive automation processes. These include:
1. Machine Learning (ML): It allows products to study data sources for pattern identification while making choices autonomously without human input. Artificial intelligence with Machine Learning applies its capabilities to recommendation systems, fraud recognition, and predictive maintenance.
2. Natural Language Processing (NLP): NLP enables AI-powered systems to interpret human language. It enables interactive NLP chatbots, voice assistants, and automated customer support.
3. Generative AI (GAI): GAI produces human-like content, including texts, images, music, and videos. Thus, making it appropriate for automated writing tasks, Generative AI chatbots, and creative design applications.
4. Predictive Analytics: Businesses can make proactive decisions in inventory management, such as forecasting and risk assessment, by using historical data in AI models.
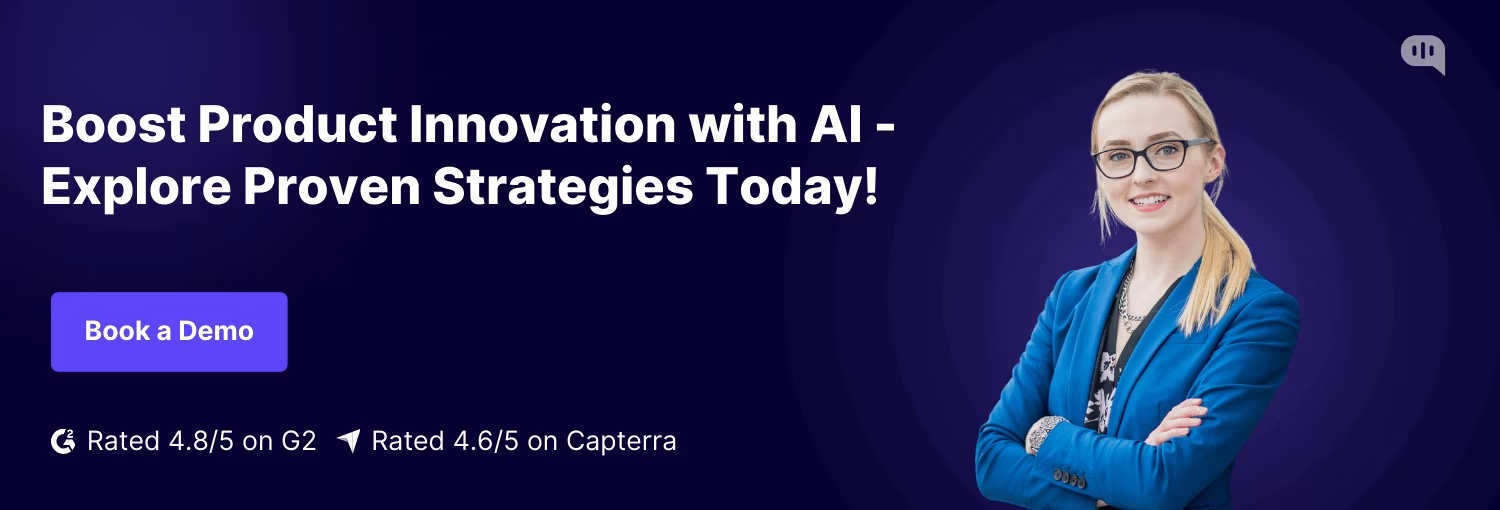
How AI Enhances Customer Experience (CX) in Product Management
Customer experience (CX) undergoes a transformative shift through AI. The technology solves several problems, including slow speeds in response times, inadequate personalization, and inconsistent service support delivery. AI-powered solutions enable businesses to:
1. Enhance Response Times: With virtual AI-integrated FAQ chatbots and assistants, they deliver immediate responses to customers, shortening wait periods and enhancing customer satisfaction.
2. Increase Efficiency: AI helps achieve operational efficiency by handling recurring operations so employees can handle complex problems and key interactions.
3. Personalize Experiences: Through behavioral analysis, AI generates customized suggestions alongside adaptable content to build an effortless and smooth user experience.
4. Predictive Analytics for Decision-Making: AI analyzes market trends and customer behavior to forecast demand and improve strategic planning.
5. AI-Powered Onboarding: By guiding consumers through product features, tutorials, and setup procedures, AI-driven onboarding lowers churn rates and increases retention.
10 Strategies for Successfully Integrating AI into Your Product Roadmap
To successfully add AI to your product roadmap, you need a clear approach that aligns AI features with business goals and enhances user experience. Here are 10 key strategies to ensure smooth integration.
1. Define Clear AI Objectives Aligned with Business Goals
To measure the effectiveness of using the company’s AI, businesses need to have the Key Performance Indicators (KPIs) set. These key metrics include:
AI Adoption Rate: The AI adoption rate represents the proportion at which businesses and their workforce deploy AI technology through daily tasks. A McKinsey report notes that 60% of organizations that have adopted AI are using generative AI, which shows an increase in AI adoption.
Operational Efficiency Gains: Operational efficiency assessment measures AI success by evaluating automation, resource optimization, and cost reduction. Meta cut 21,000 jobs in 2023, focusing on AI operational efficiency, leading to a 201% increase in net income and a 178% stock surge.
Customer Satisfaction (CSAT): An AI-based metric of customer satisfaction measurement in terms of improvement in personalized services, speed of response, and service quality improvement. For example, AXAA – Axis Bank’s AI voice assistant – handles about 12-15% of customer calls with 90% accuracy, sealing off call response times and service quality.
2. Assess Feasibility and Market Readiness
Organizations must evaluate their capacity to adapt to AI before seeing benefits from business investment in this technology. This involves assessing:
Data Infrastructure: To add AI functionality to the system, you must create a solid framework for data storage, processing, and acquisition. Using AI systems enhanced Cleveland Clinic’s hospital procedures, allowing it to grow its operating margin by 0.4% despite rising costs.
Technical Expertise: The organization needs trained experts to properly create and run AI solutions. JPMorgan achieved instant document review instead of 360,000 hours annually through their AI system called COiN.
Cultural Readiness: Encouraging an innovation-driven mindset helps teams adapt to AI-driven workflow changes. A Deloitte study shows that 91% of companies believe AI will enhance performance.
To accommodate evolving business needs, AI models should be designed for scalability through:
Modularization: Artificial Intelligence enables easy updates to its structure when a project needs modification.
Cloud Integration: The cloud integration system provides maximum flexibility and supports artificial intelligence technology and data storage needs.
3. Selecting the Right AI Technology Stack
Choose AI models and platforms like AI-powered email ticketing to automate support workflows and enhance efficiency. The selection process should focus on:
AI Models: Depending on your business needs, various models can be employed:
Generative AI (GAI): GAI technology generates new text, images, and music, boosting creative output and customizing results.
Large Language Models (LLMs): Support services and content development receive better text analysis and production through Large Language Models, especially GPT and BERT.
Machine Learning Frameworks: The machine learning industry offers the TensorFlow and PyTorch platforms to develop and use AI models effectively.
AI Development Platforms: Hugging Face and Google Vertex AI simplify AI development by giving businesses complete tools to build, train, and release models to production.
Business systems need to work smoothly with AI technology for optimal business output. Key aspects include:
Compatibility: The AI tools should integrate effectively with CRM systems, helpdesks, and other business platforms so operations remain uninterrupted.
Data Flow: A secure and fast connection between AI models and their data sources lets models operate better when handling new information for decision support.
Scalability: The selected AI software must handle rising data volumes and updated business operations without interrupting daily work.
4. Develop a Robust Data Strategy
AI systems achieve success only when they work with good-quality data. Ensure all data is precise while following legal requirements such as GDPR and HIPAA regulations.
Essential data attributes include:
Accurate and Reliable: Keeping data consistent and correct assures the AI system will not produce faulty results from information. Tesla’s autonomous driving AI relies on high-quality data from over 3 billion miles of real-world driving.
Comprehensive: The system records all needed information from diverse aspects to give AI models appropriate data for analysis..
Unbiased: To achieve unbiased results, one should use diverse approaches to gather and handle data.
A robust data engineering team should always validate and normalize data while removing irregularities to keep data accurate. Following the ISO/IEC JTC 1/SC 42 standard, organizations find guidance for keeping AI application data sets reliable.
Companies must respect data protection laws to build customer trust and follow official rules. Key regulatory aspects include:
Data Minimization: Collect only basic data that enables an AI system to do its required tasks.
Consent Management: Ensuring explicit user consent before collecting, processing, or utilizing personal data and GDPR compliance is essential. Amazon received an $877 million fine for its AI system privacy violations.
Transparency: Clearly communicating how data is used to build trust among users and stakeholders. AI credit scoring systems have made lenders more equitable by fixing past errors in traditional evaluation methods.
5. Implement AI in Pilot Projects Before Scaling
Testing AI technology through pilot projects allows businesses to test and enhance models before they go live in their entire system. Smaller test implementations help companies find problems and perfect their AI systems.
Risk Mitigation: By observing AI systems, pilots help producers find problems so that companies can adopt them safely across their operations. Before deploying AI nationally, McDonald’s tested it in drive-through locations to reduce mistakes and enhance how quickly orders were made.
Operational Refinement: AI tests performed in business operations help companies both improve their product accuracy and make their systems work together better. Dropbox released 16% of its staff to reassign to AI projects to enhance AI development and product transformation.
Cost Efficiency: The planned stage-outs handle resources better by guaranteeing that AI systems bring practical results. Businesses can use AI tests to direct budget usage effectively.
6. Build a Cross-Functional AI Team
Reaching successful AI implementation requires building a proper organization that links AI development with overall operations. The customer success team learns AI skills better through teamwork with multiple functional areas while creating solutions that benefit customers.
A strong AI team ensures:
Interdisciplinary Collaboration: Apple’s AI team, which consists of engineers, UX designers, and linguists, works together to develop Siri updates.
Breaking Down Silos: JPMorgan and its AI team linked various financial analysis units into one system, which decreased mistakes in reviewing credit agreements.
Strategic Alignment: AI product managers bridge the gap between business needs and technical execution, ensuring ROI.
7. Prioritize Ethical AI and Transparency
Companies should use ethical AI methods while maintaining open information to build trust and ensure responsibility. AI systems require training on various data types to produce inclusive and unbiased results.
The system containing facial recognition technology proved to be an error for dark-skinned individuals, which shows the need for better ideas in AI. Through research, Joy Buolamwini forced tech companies to change their algorithms to reduce biases.
To enhance transparency and accountability in AI-driven decision-making:
Through Explainable AI (XAI) technology, users receive explanations about AI decision-making that build trust and maintain fairness standards.
The Algorithmic Justice League works to make algorithms more open while fixing automated tools that show bias in their decisions.
AI algorithms examine different banking records to improve lending decisions and stop discrimination in normal credit evaluations.
8. Leverage AI for Customer Experience & Personalization
Businesses use AI technology to enhance customer experiences by offering tailored, efficient, and interactive relationships. Predictive analytics and automation improve how businesses contact customers, which increases their satisfaction and makes them stay loyal.
Businesses are using AI technology to improve different aspects of customer service.
AI-powered automation: Through its automation technology, Tesco’s AI-enhanced Clubcard systems helped retain customers better by 12%.
Balancing AI and human touch: Amarra keeps AI tools running their inventory tasks while having people handle customization orders in their formalwear business.
Personalized shopping experiences: Companies see 35% better sales bookings when their e-commerce websites provide AI-based customized recommendations to customers.
9. Implement AI Testing, Quality Assurance, and Monitoring
Ensuring the reliability and effectiveness of AI systems requires a structured approach to testing, quality assurance (QA), and continuous monitoring. AI models must be regularly tested and refined to maintain performance, accuracy, and security.
Businesses can enhance AI reliability through:
Automated Testing: AI automates repetitive testing tasks, enabling continuous testing, real-time feedback, and faster release cycles. AI reduces software testing costs by 20%, accelerating release cycles.
Ongoing Model Updates: Continuous model retraining stops accuracy loss from happening when data behavior changes. Amazon conducts normal AI training sessions with Alexa to develop better language processing.
Continuous Monitoring: Real-time data helps identify performance issues and track security updates, maintaining reliable performance while eliminating unfair behavior. AI systems used by the banking industry receive routine checks to stop unfair practices in loan approval procedures.
10. Plan for AI Scalability and Future Growth
Businesses must ensure AI technology grows and adjusts according to new technology to thrive in their market and keep expanding. AI is built flexibly, it can easily accept newer technologies such as IoT, blockchain, and AR/VR to improve business procedures.
Organizations need these main strategies to improve their AI systems across many locations:
Interconnected Systems: Successful AI must combine with future technology platforms to improve business automation tools and better connect with customers. Using Artificial Intelligence to streamline supply chain operations reduces operating costs by 15%.
Customer Experience Automation: AI-powered chatbots handle 80% of everyday customer questions through automated systems to cut company hiring expenses.
Data-Driven Decision-Making: AI analytics in finance helps institutions like Goldman Sachs make data-backed investment decisions.
Challenges and Risks in AI Product Management
The AI product management domain contains multiple operational and security concerns that organizations must resolve for proper execution and system functionality. Key areas of concern include:
Common Pitfalls in AI Integration
Slow Adoption: Even with significant budget allocation by most organizations, AI implementation has made little progress. For instance, 58% of Australian firms reported that AI technologies did not meet their expectations, leading to a slowdown in further implementation efforts.
Lack of Customer Experience (CX) Alignment: AI solutions that do not match customer needs will lead to unsatisfactory user experiences due to misaligned Customer Experience (CX). The success of AI depends on maintaining customer satisfaction through positive CX improvements.
AI Resistance: Employee apprehension regarding AI is prevalent; a survey revealed that 71% of U.S. employees have concerns about AI’s implications, with 75% fearing job obsolescence and 65% uneasy about AI replacing their roles.
AI Governance & Compliance
Managing AI Ethics: Developing ethical AI systems depends on following principles to stop damage and achieve equality. The Artificial Intelligence Act from the European Union shows how regulatory forces aim to maintain ethical standards across AI creation and implementation.
Security Risks: The security vulnerabilities of AI systems include defense against adversaries who perform attacks while exposing data to breaches. Binding security solutions remain essential because they defend critical information and safeguard the complete system integrity..
Global Regulations: Businesses must understand AI regulations in different regions to comply with international laws. For example, the U.S. government has developed the AI Bill of Rights to provide ethical guidelines on AI technology deployment and use.
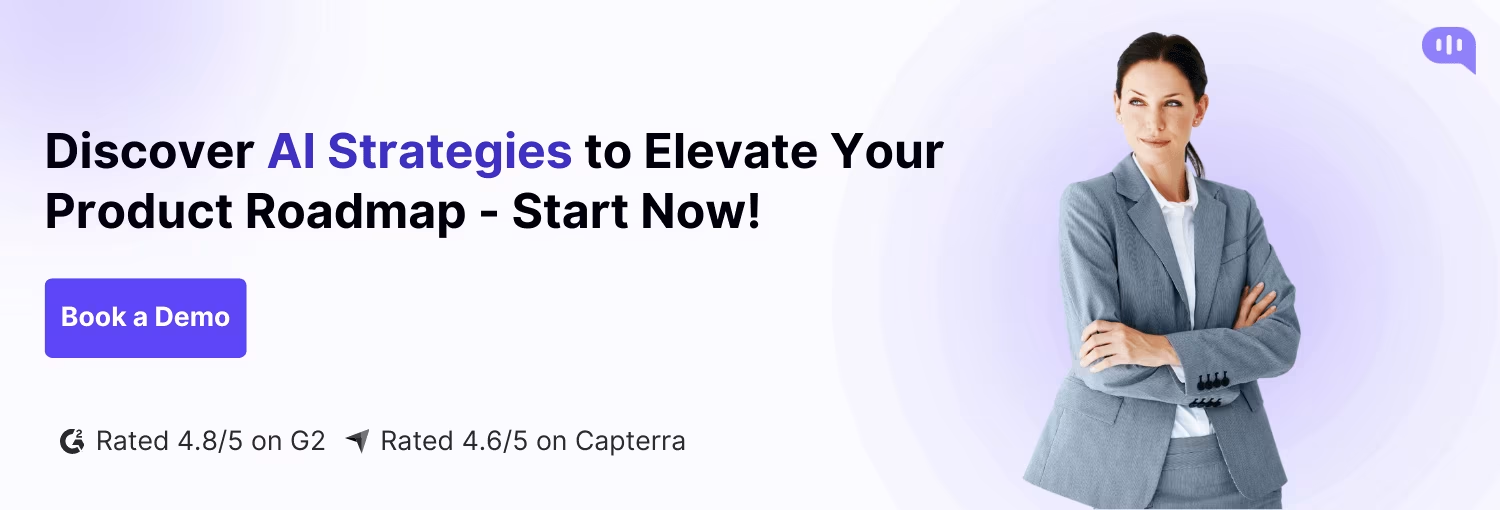
Risk Mitigation & Future AI Trends
AI Audit Frameworks: Provide mechanisms that enable functional testing and ethical compliance of AI systems. TRUST framework provides extensive testing procedures and continuous assessment to develop trustworthy Artificial Intelligence systems.
Bias Detection Tools: Deploying tools to identify and mitigate biases in AI models is crucial for fairness and equity. Continuous evaluation helps prevent discriminatory outcomes.
Regulatory Policies: Organizations must develop proactive relationships with regulators who set and adapt universal policies to ensure AI adoption follows ethical standards and legal requirements. A global network called the International Network of AI Safety Institutes works toward resolving national security issues involved with artificial intelligence.
Return on Investment (ROI) Challenges
The ROI assessment of AI initiative becomes complex because of its initial high costs, integration difficulties, and maintenance requirements. Organizations must weigh AI’s advantages against these points to acquire lasting value. Strategic planning, strong governance systems, and ethical considerations create the foundation for responsibly implementing AI at its full potential.
The Bottom Line
Implementing AI product management requires organizations to follow specific formal approaches and strategic guidelines. User trust maintenance and open transparency become possible through the requirement for AI scalability together with ethical operations and regulatory standards.
The implementation of AI for product leaders requires repeated stages of change. Businesses that begin with test-run AI projects and improve these algorithms along with responsible implementation will achieve sustained success. When executed strategically, an AI system establishes operational excellence and innovative customer interactions to fuel business growth throughout the AI-operated future.
As the Head of Growth, Marketing & Sales, Yogesh is a dynamic and results-driven leader with over 10+ years of experience in strategic marketing, sales, and business development.