Updated on February 27, 2025
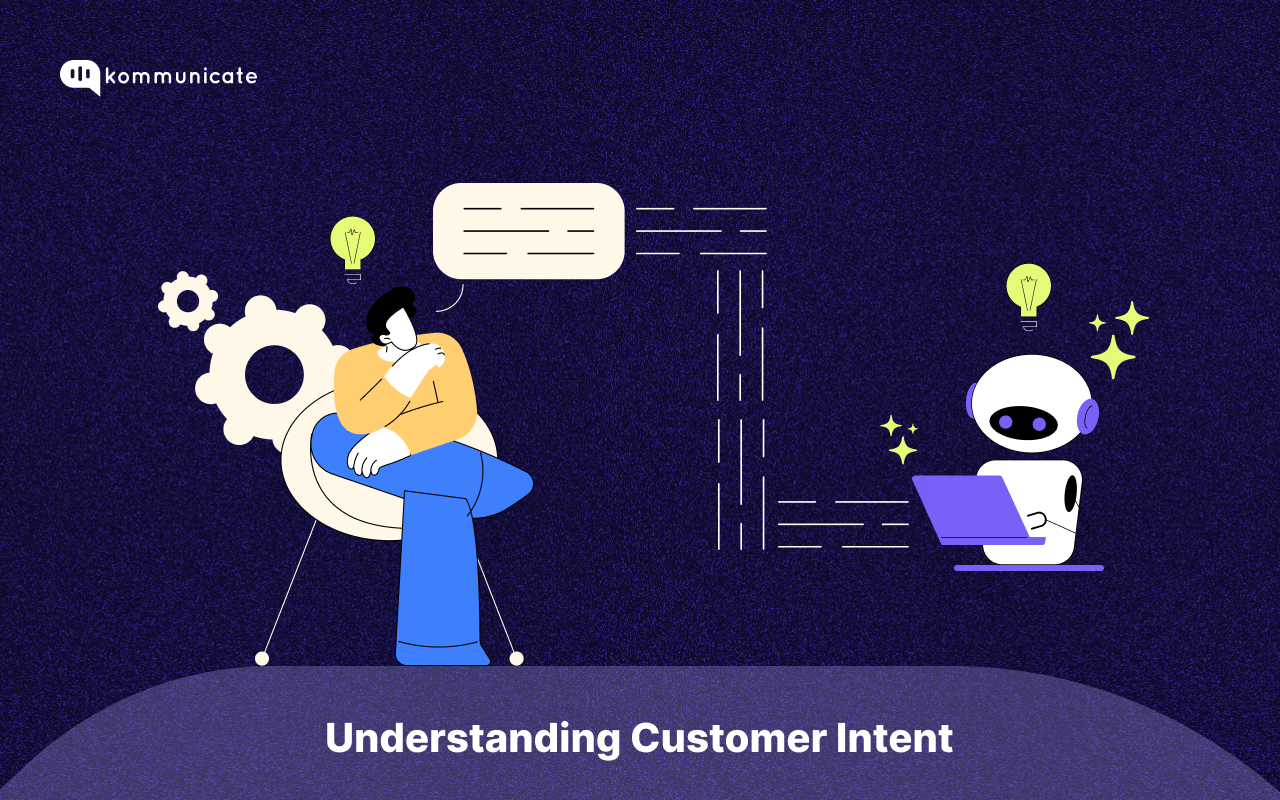
Customer intent optimization or buyer’s intent analysis refers to the customer actions or behaviors traditionally used by businesses to identify customers who want to purchase particular products or services. However, as the relationship between consumers and brands has evolved, so has the meaning of customer intent marketing strategies.
Today, customer intent analytics measurements map the consumers’ journey through the product experience, marketing, and customer support automation funnels in a business. Simply put, our current definition of customer intent tracking encompasses.
So, why is this modern version of customer intent important? Let’s understand the nuances.
What is the Importance of Measuring Customer Intent?
70% of CX leaders invest in AI-driven customer analytics solutions automatically capturing and measuring customer intent data. As many as 71% of customers want AI-powered personalized customer experiences; these measurements drive customer retention and boost online sales.
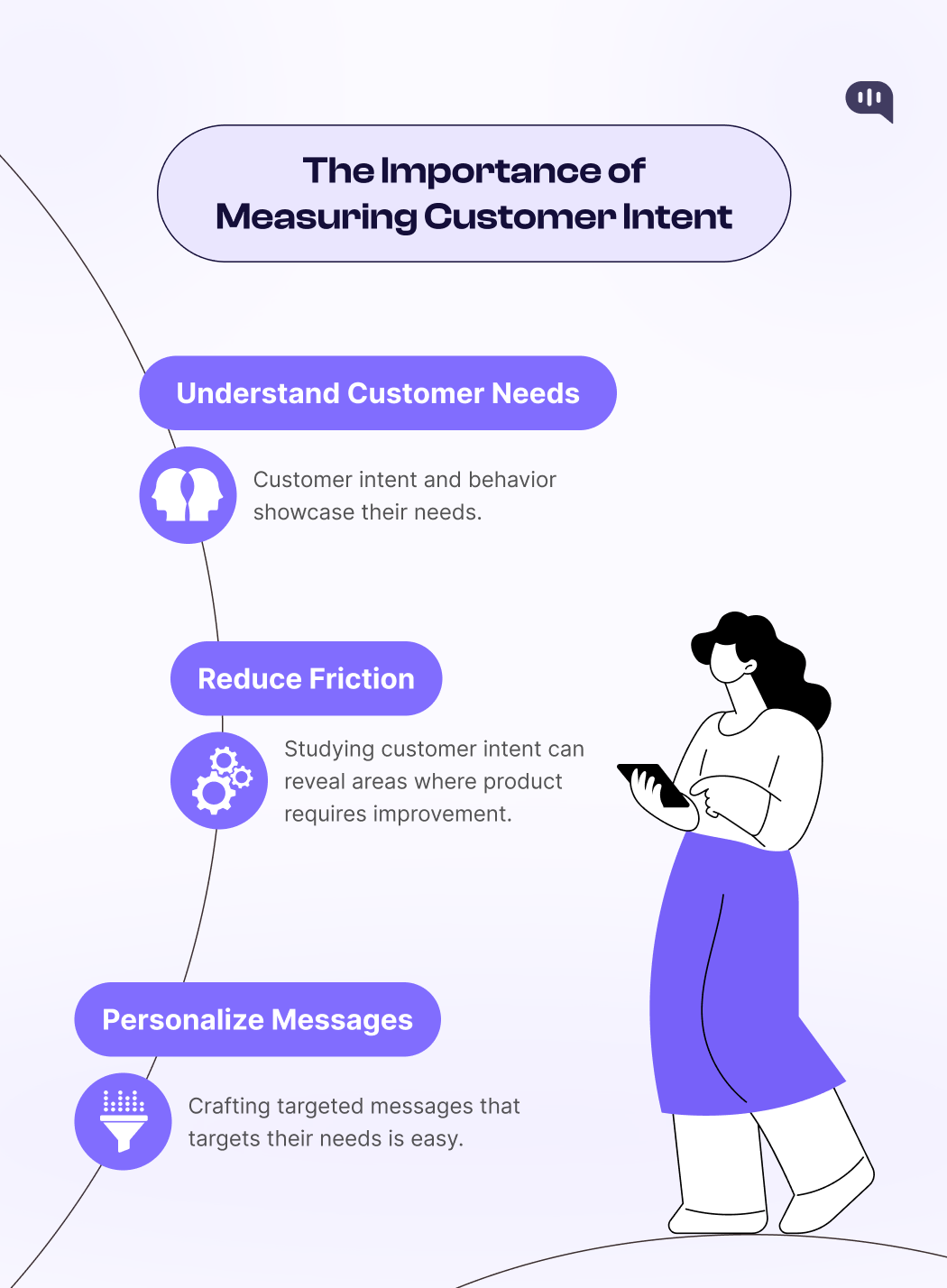
Practically, these measurements are used to:
- Understand Customer Needs: Customer intent and behavior showcase their needs. For example, if a customer keeps enquiring about a particular feature, it might be an essential requirement for their business.
Understanding these cues helps you drive more sales through target, personalized messages. - Reduce Friction: Studying customer intent can often reveal areas where your product requires improvement. For example, If many customers complain about an integration, a more straightforward method may help.
- Personalize Messages: When you understand the customer, crafting personalized messages targeting their needs is reasonably straightforward. This helps to create better support processes and drive sales.
However, AI-based customer intent measurement takes work. Tracking user behavior is challenging because it has many dimensions, from website visitor analytics to detailed conversations with AI chatbots for customer engagement. This is where advanced data analysis with AI can help. Let’s see how that works at scale.
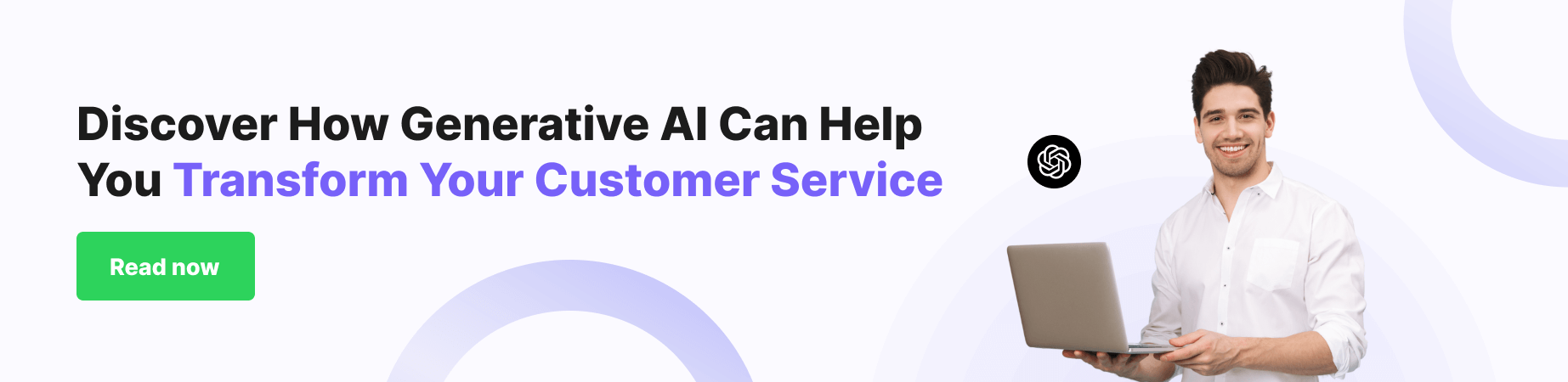
How Can You Use Generative AI to Understand Customer Intent?
There are two aspects of customer intent that generative AI tracks. These are:
- Non-Conversational Data: Customer intent is captured through data around the pages your users visit or the features they use most frequently.
- Conversational Data: Sales calls, chatbot conversations, and support tickets are all sources of conversational data that can be used to measure customer intent.
Let’s examine how generative AI is used to identify intent in both cases.
Non-Conversational Data
Most businesses use suites like GA4 to track customers through their websites. Software that tracks users’ clicks and visits has been around for years. AI can analyze the large-scale of data to get:
- Conversion Efficiency: If you see every touchpoint individually, you can identify the touchpoints that drive the most conversions for your business. This helps you identify high-intent customers.
- Engagement Patterns: Customers who engage with your product or content plan to buy or upgrade their services. This is also a measure of whether someone is high-intent or not.
- Friction Points: If customers often complain about a particular feature or page, it might be time for a change. Analyzing user actions at scale can let you understand the bottlenecks your users face when they use your products or services.
Now that we understand how generative AI uses non-conversational data let’s focus on conversational data instead.
Conversational Data
While the data around clicks and funnels come in numbers, conversational data is almost always text. This leads to a large-scale problem with massive textual data that doesn’t translate into meaningful customer intent measurements.
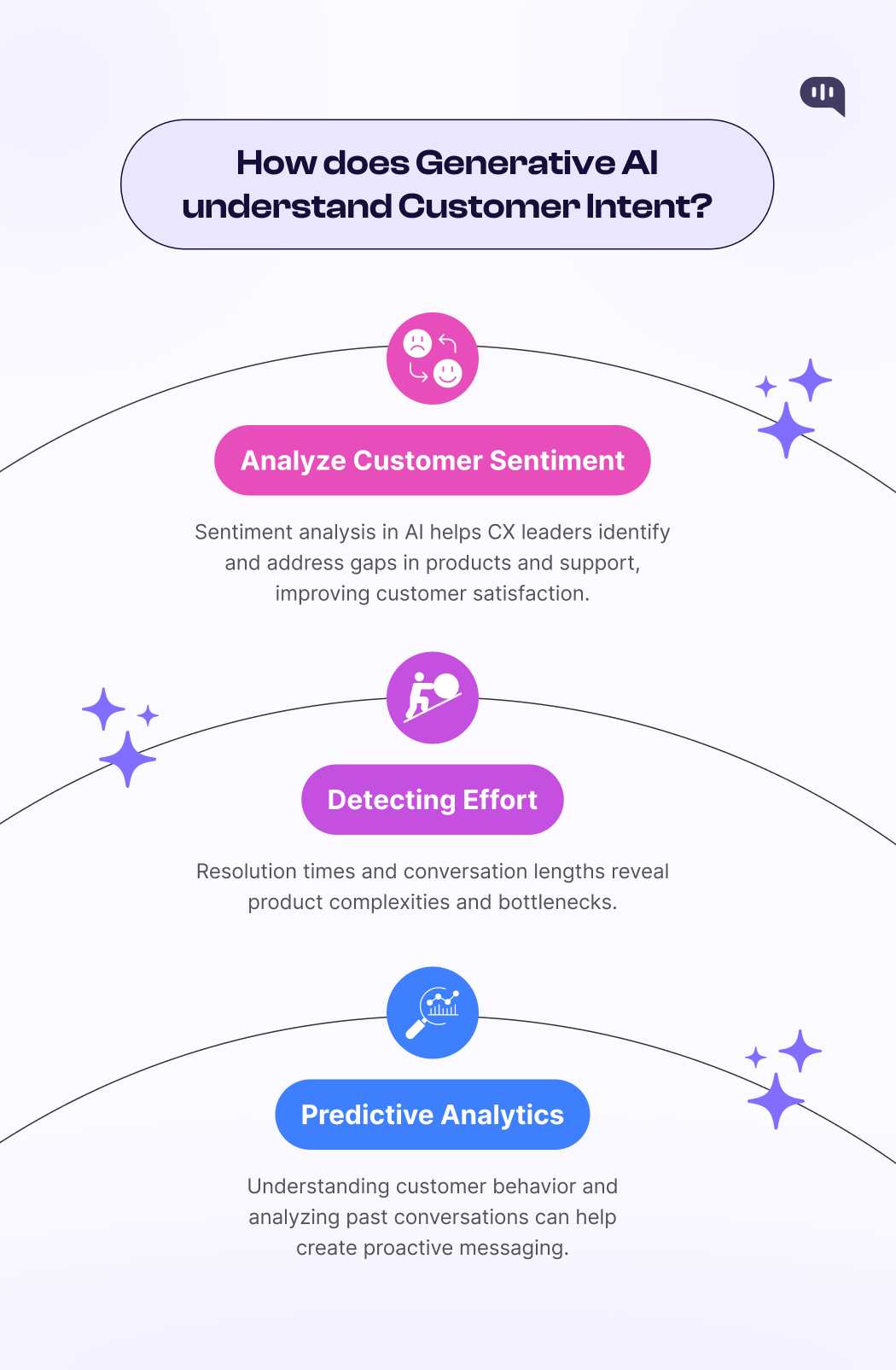
Generative AI solves this problem using AI-driven intent recognition and advanced natural language processing (NLP) systems that capture crucial information from customer conversations with AI chatbots.
Essentially, generative AI chatbots can:
- Analyze Customer Sentiment: Sentiment analysis is a standard ML functionality built into generative AI applications. It can understand if a customer is happy, sad, or frustrated at one of the services. By identifying these areas of conversation, this analysis can help CX leaders bridge gaps in their product and support systems.
- Detecting Effort: Resolution times and conversation lengths can help you understand complex parts of your product or service. This can help you identify common bottlenecks for adoption. For example, If a customer has to ask five times to resolve a problem, there might be issues with that particular touchpoint.
- Predictive Analytics: Understanding customer behavior and analyzing past conversations can help create proactive messaging. For example, AI can anticipate the servicing needs of a consumer based on the history of their support tickets.
These three methods are essential to understand customer behavior in the modern age. However, generative AI doesn’t stop at measurement. It’s also integral to creating strategic actions based on these intents.
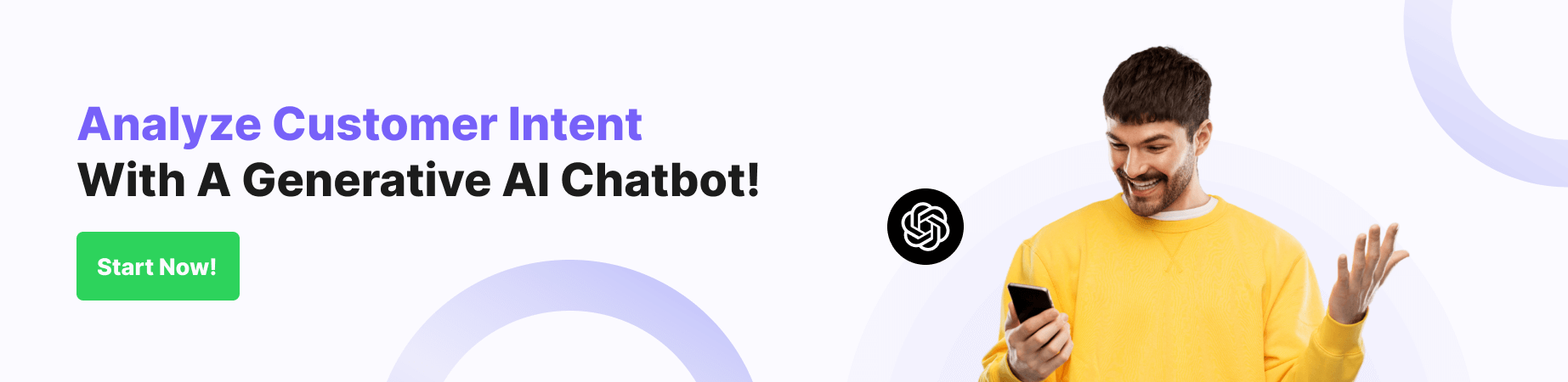
How to Turn Customer Intent into Actions Using Generative AI?
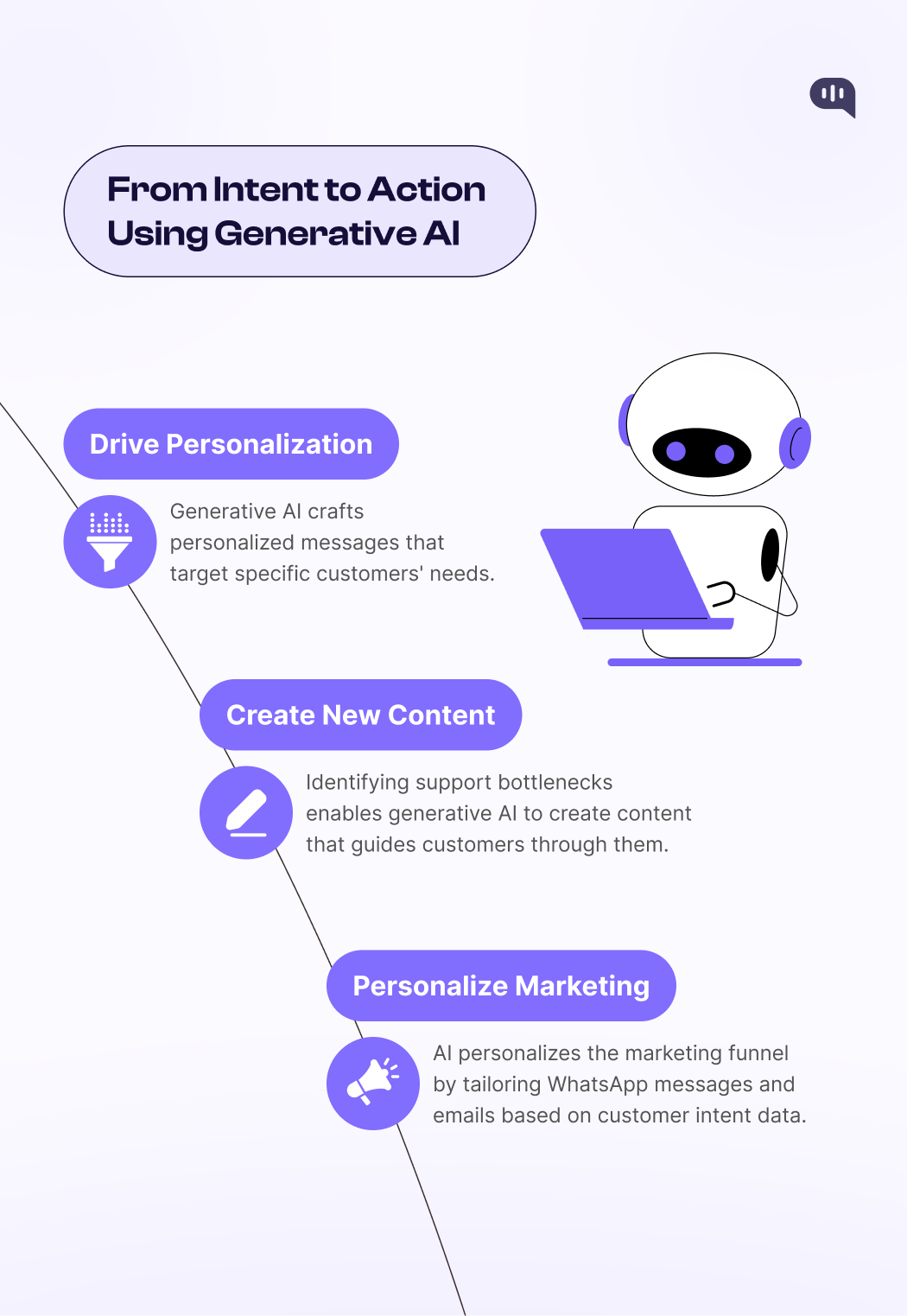
When you have large-scale customer intent data, you must turn it into actions that retain and convert your prospects. This is also done through generative AI, which can:
- Drive Personalization at Scale: Generative AI can craft personalized messages that target specific customers’ needs. Since you already have data about how the customer approaches your products and services, generative AI can offer personalized recommendations that help customers take action.
- Generating New Content: When you identify bottlenecks within your support processes, generative AI can develop new content that helps customers through these places. This can include Knowledge Base articles and tutorials that help customers through a particular product feature or marketing emails that guide users to the next feature they might find interesting.
- Creating Personalized Marketing Journeys: AI can drive personalized experiences that cover the whole marketing funnel. Using customer intent data, generative AI can personalize the texts you send through your Whatsapp chatbot and tailor your emails to the customer’s use case.
With customer intent data, generative AI can drive personalization and increase your retention and sales at scale. Since generative AI can understand and adapt to customer needs, it can drive significant ROI for businesses.
Transform your customer support with Kommunicate’s
AI-powered email ticketing—resolve queries faster than ever!
Also Read: Conversational AI vs. Generative AI: Understanding the Differences
Also Read: Implementing Generative AI – A Step-by-Step Guide for Product Managers
Also Read: How Large Enterprises Harness Generative AI for Customer Engagement
Final Thoughts
Customer engagement has changed over the past few years. With more customers expecting personalized services and proactive support, driving better customer experiences requires an in-depth understanding of customer intent.
Customer intent measurements have also evolved to encompass the entire customer journey. Customer intent measurements are now used to address customer needs, create frictionless product journeys, and personalize messages.
Since customer intent measurement requires large-scale data analysis, generative AI can help. It can analyze the clicks on your apps and websites and conversations with your prospects.
These capabilities also allow generative AI applications to drive personalization, generate content, and improve customer experience.
Maximizing customer experience using customer intent data can improve sales and retention, driving significant ROI for businesses.
As the Head of Growth, Marketing & Sales, Yogesh is a dynamic and results-driven leader with over 10+ years of experience in strategic marketing, sales, and business development.